Data scientists are some of the most in-demand professionals on the market. A LinkedIn Workforce Report in 2018 found 151,000 unfilled data scientist jobs across the United States, with “acute” shortages in San Francisco, Los Angeles, and New York City. And the demand for data scientists is only rising. The number of data scientist positions is expected to grow by 15% between 2019 and 2029.
With such a scarcity of data scientists, many companies face a critical skills gap that cannot be closed by hiring. The costs for such a gap can be significant. In a data-driven marketplace, companies that lack the personnel to maximize the value of big data operate at a competitive disadvantage.
In this three part series, we will discuss how organizations can unlock the full potential of big data by closing the data science skills gap. This is Part 1 of the series. In this installment, we will explain and contextualize today’s data science skills gap, and how it impacts data-driven companies.
Companies Need Data Scientists to Thrive in a Hyper-Competitive Market
With 90% of the world’s data created in just the past two years, basic data analytics are no longer sufficient to anchor the business operations of most leading companies. That’s where data scientists come in. Data scientists use scientific methods, procedures, disciplines, and frameworks to extract key insights from an ever-growing volume of both structured and unstructured data.
Data scientists combine fields such as machine learning, artificial intelligence, information science, statistics, and computer science. They often focus on converting big data into usable information, and charting optimal company operations. Businesses need data scientists to produce advanced analytics and predictive insights. Data scientists help forecast if changes will be effective before implementing them.
Applications for data science are vast and fast-evolving. Data science powers countless business use cases, from targeting ads, to optimizing shipping routes, to detecting fraud, to identifying diseases, to leveraging investments, and much more. This is why data scientists are in such high demand. Leading companies need data scientists to thrive in the hyper-competitive era of big data.
Why, then, is there such a data science skills gap in the market? There are two primary reasons: training and utilization. Here’s why.
The Data Scientist Shortage: A Lack of Training, Education, and Identification
Even though data scientist is one of the fastest growing professions, not enough workers possess the requisite skills to perform the role. Data scientist is a multi-disciplinary position that requires a background in computer programming, statistics, data modeling, and a number of other technically involved fields. In order to cultivate these talents, workers must either seek out an educational pathway that incorporates these disciplines, or engage in supplemental or self-directed study.
Many educational institutions have yet to formalize data science degrees and curricula. At most institutions, the disciplines that comprise data science are unintegrated. Students who major in computer science are trained in algorithmic construction, while those who major in applied math acquire expertise in statistical modeling. Both knowledge domains are key for data science, but they are not necessarily taught together.
This lack of a traditional educational pathway for data science does not necessarily represent institutional apathy. Rather, the gap reflects the incalculable explosion of data in the past decade. Before the rise of big data, data science was a somewhat academic discipline, rife with thought experiments and philosophizing. But advancements such as smartphones increased data collection enormously. And educational institutions have been playing catch up ever since.
Some educational institutions have made strides in recent years. Leading universities such as UC Berkeley have added data science as an undergraduate discipline. Others, such as Stanford, offer graduate tracks in data science. But many institutions offer no formal end-to-end pathway for data science study. The result is that those who want to train in data science must seek such education on their own pathways. And this becomes its own vicious cycle. Students who are technologically inclined can just jump into a field like software engineering much faster.
Many companies hiring data scientists require undergraduate and graduate degrees in related fields, such as math, physics, or computer science. However, those who seek these rigorous degrees can also pursue roles in finance, programming, or another lucrative profession. And by requiring such educational credentials, companies exclude applicants who have the skills, but not the piece of paper. Data Science Bootcamps have emerged to fill the market need, but these courses are not yet as popular as Coding Bootcamps.
The result of this lack of training, education, and ability to screen talent has resulted in a significant shortage in data scientists.
Poor Utilization: Data Scientists Spend Their Time on Inexpert Tasks
But the shortage of workers is not the only factor in the data science skills gap. The other core issue is that data scientists often waste their time doing manual tasks as opposed to using their sought-after skills.
Say the phrase “data scientist,” and you might conjure an image of someone concocting complex machine learning algorithms. After all, many data scientists spent years earning MAs and PhDs in rigorous disciplines. They have mastered an alphabet soup of programming languages, from R, to SQL, to Python.
The core value of data scientists is directly tied to this expertise, through the generation of models, algorithms, and business insights. However, many data scientists will tell you that on a day-to-day basis, devoting uninterrupted time to these projects is difficult. Data scientists spend many of their hours performing rote tasks that fail to put their advanced skills to good use.
Consider the numbers. Studies have shown that most of a data scientist’s time is spent cleaning data. In fact, gathering and cleaning data accounts for about 41% of a data scientist’s time, whereas building and running models accounts for only about 31%. That’s the sad truth: many data scientists spend more time performing grunt work than they do producing insights.
This causes a problem within a problem. Not only are data scientists in short supply, but they also waste their precious time performing tasks that do not require advanced skills. However, by eliminating this grunt work, data scientists can focus on delivering the valuable insights they were hired for.
And that’s where data management platforms come in.
Coming Soon – Closing the Data Science Skills Gap (Part 2): Data Management Platforms
Stay tuned for in the coming days for Part 2 of our series! Find out why data management platforms are critical for eliminating manual tasks, so data scientists can focus on generating the expert insights they were hired for.
Minimize the firefighting. Maximize ROI on pipelines.
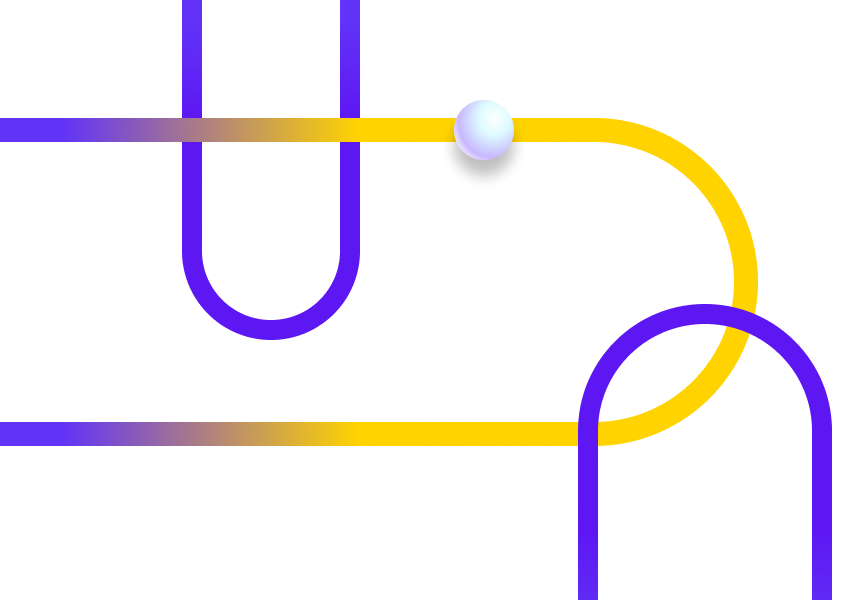
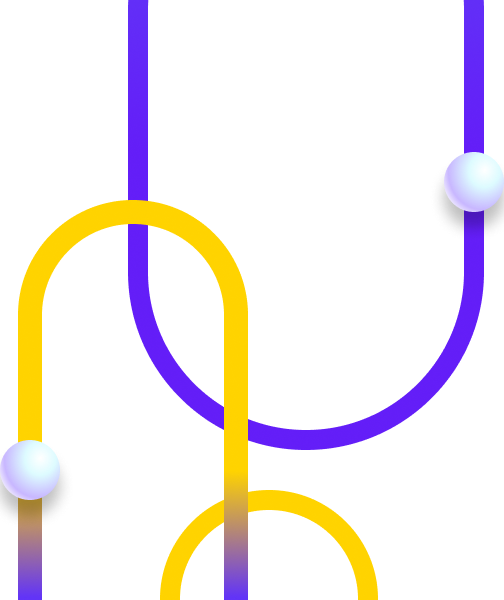