2022 is here and the data management landscape is changing faster than ever before with new trends and emergent technologies.
As the data mesh, self-service, and data citizens disrupt the current landscape, the core functions of data engineering will remain critical. Amid these fast-moving developments, companies will have to make key decisions in 2022 about the modern data stack, personnel, and so many other emergent possibilities.
That’s why we developed a new eBook: Data Management 2022: Trends, Technologies, Teams, and Organizations. This 22-page guide lays out everything you need to stay ahead of the curve and optimize your data management processes for 2022.
Here’s an excerpt from the eBook about six key trends and technologies that will drive data management in 2022, including Reverse ETL, the Analytics Engineer, MLOps, Active Metadata, Data Governance, and Data as a Product.
1. Reverse ETL
Since the advent of cloud data warehousing, business users have leveraged BI dashboards to make decisions and drive initiatives.
However, the BI process remains full of bottlenecks and inefficiencies, from measuring the wrong metrics, to slow roll outs, to low utilization. BI dashboards also do not allow business users to manipulate and operationalize data in business processes.
Reverse ETL bypasses dashboarding altogether by pushing data directly into 3rd party systems (CRM, CDP, ERP, etc.) for direct usage. In 2022, reverse ETL will become a key component of the modern data stack for many companies.
ETL and ELT both transfer data from third-party systems, such as business applications (Hubspot, Salesforce) and databases (Oracle, MySQL), into target data warehouses. But with reverse ETL, the data warehouse is the source, rather than the target. The target is a third-party system.
In reverse ETL, data is extracted from the data warehouse, transformed inside the warehouse to meet the data formatting requirements of the third-party system, and then loaded into the system for action.
By pushing data back into third-party systems such as business applications, reverse ETL operationalizes data throughout an organization. Reverse ETL enables any team, from sales, to marketing, to product, to access the data they need, within the systems they use. The applications of reverse ETL are numerous, but some examples include:
- Syncing internal support channels with Zendesk to prioritize customer service
- Pushing customer data to Salesforce to enhance the sales process
- Adding product metrics to Gainsight to improve the customer experience
- Combining support, sales, and product data in Hubspot to personalize marketing campaigns for customers
What teams really want is to access data within the systems and processes that they’re already using. This is exactly what reverse ETL enables you to do. With reverse ETL, business users can actually harness data in an operational capacity. Teams can act on the data in real-time via change data capture (CDC), and use it to make key decisions, while leveraging BI dashboards as supplementation.
2. Analytics Engineer
As the gap between business and technical teams continues to grow, a new role has emerged to bridge the divide.
The Analytics Engineer is a relatively new position that fills the gap between data engineers and data analysts. The role has grown in popularity recently, and in 2022, that trend will continue to grow.
Analytics Engineers build data models based on business requirements to produce analytics for stakeholders and teams. Unlike data analysts, analytics engineers do not analyze data; they transform, test, deploy, and document data for business users. Analytics engineers apply version control, CI/CD, and other SE best-practices to the analytics code base to ensure the quality, consistency, and value of data. They are, in short, the role that closes the chasm between business and IT.
And this is what makes Analytics Engineers so important for data-driven companies. MPP databases have abstracted away the difficult computer science problems, democratizing the ability to process large amounts of data. Today, what companies need is a “first-hire” role that can set up and activate the modern data stack (more on this topic later).
An Analytics Engineer is someone who is technical enough to launch a cloud data warehouse and data pipelines, but also someone who has the business sense to translate and materialize the analytics requirements of users. Analytics Engineers can set up basic data infrastructure and visualizations — an appealing prospect for companies that do not yet need deep engineering firepower.
3. MLOps
In 2022, advances in data management will enable teams and companies to unlock new capabilities.
This includes functions such as MLOps. MLOps is a set of practices and protocols that deploys and maintains machine learning models in production. Just as DevOps improved software engineering, and DataOps improved data engineering, MLOps is improving data science.
MLOps applies to the entire ML lifecycle, from data gathering, model creation, CI/CD, and orchestration to deployment, health, diagnostics, governance, and business metrics. Key components of the MLOps cycle — such as data gathering and data transformation — are streamlined by data management platforms.
4. Active Metadata
Metadata has always played a key role in data management. However, with the emergence of the modern data stack, metadata management has lagged behind other components.
Companies can set up a data warehouse in less than an hour, but building out data cataloging and other metadata-centric solutions often takes months. Meanwhile, friction between cross-functional teams, from engineers to salespeople, has made metadata management more important than ever.
Enter active metadata. Active metadata refers to data that defines data, including data about what happens to the data. Combining technical, operational, business, and social metadata, active metadata is capable of driving faster, action-based data management deployments, from alerting to operationalizing insights.
“One of the strong themes for Gartner is the idea of active metadata,” TopQuadrant CEO, Irene Polikoff, acknowledged in a recent article. “One aspect of that is it’s directly actionable; it’s actually used in real-time by operational systems to do various things.”
Active metadata enables quicker and more scalable solutions for data cataloging, lineage, discovery, and governance, enhancing the speed and flexibility of the modern data stack. That’s why Garnter recently retired its Magic Quadrant for Metadata Management Solutions and introduced the Market Guide for Active Metadata. And in 2022, this trend will continue to become more pronounced.
5. Data Governance
In 2022, a governance framework will no longer be a passive set of protocols, but a dynamic factor in analytics and decision making.
For the past decade, companies built data governance frameworks for data privacy, regulatory compliance, and other areas of risk management. But now, with the advent of active metadata, data governance can also function in an operational capacity.
Data governance frameworks that leverage active metadata and operational functionality will enhance data modeling, data stewardship, AI/ML, and more across an organization. Active metadata will also enable data governance frameworks to offer transparency of data profiles, classification, quality, location, lineage, and context. Moreover, advances in data intelligence will democratize data while ensuring security.
Governance solutions will facilitate real-time communications between different systems in a data fabric. New “metadata graphs” will enable more granularity in data lineage and BI, anomaly analysis, and impact analysis. Additionally, governance frameworks will leverage smart inferences based on active metadata to automate compliance measures for similar sources and users.
The days of passive data governance are over. In 2022, active metadata will make data governance an operational force.
6. Data as a Product
With the introduction of the data mesh (more below), the concept of “data as a product” has boomed in popularity.
A “data product” is typically a digital product or feature that uses data to achieve its end. But data mesh also discusses the productization of data — the application of the product life cycle to data deliverables.
In this new paradigm, data assets are treated as products, and data consumers are treated as customers. This framework applies product thinking to datasets, and ensures that they retain the discoverability, security, explorability, and other facets needed to remain leverageable entities.
Can't miss insights
Minimize the firefighting. Maximize ROI on pipelines.
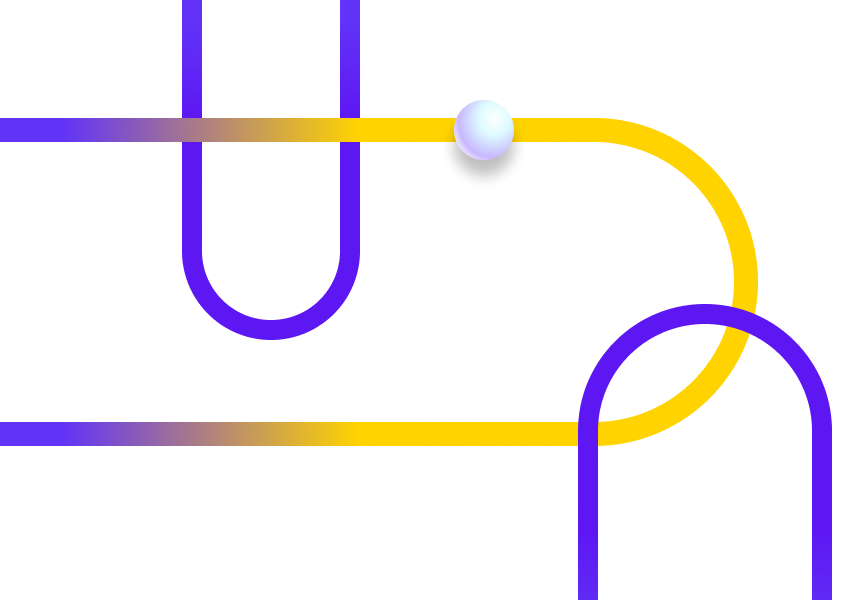
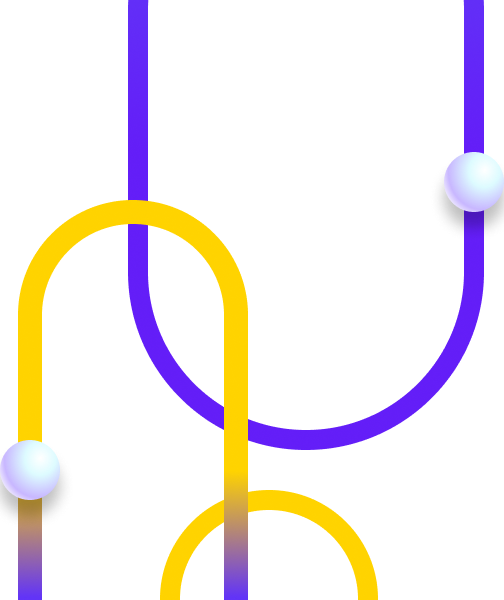