What Is Change Data Capture (CDC)?
Change data capture (CDC) is a process that tracks and identifies changes in data from various data sources such as databases and data warehouses.
This involves capturing these changes in real-time and delivering them to destination systems, ensuring data integrity and consistency.
Simply put, CDC looks for shifts in a database, and when it finds one, it records it. This record is later stored either in the same database or in external applications.
CDC enables organizations to efficiently manage data across different deployment environments by ensuring that any updates, inserts, or deletions are reflected accurately in downstream processes.
The best thing about CDC is by maintaining up-to-date data, it supports real-time analytics and operational efficiency, allowing data analysts to indulge in the most accurate real-time data science and analytics.
CDC creates a smooth flow and increases the system’s reliability which is especially crucial in cloud architectures or a data warehouse in general, where there is constant flow and integration of data.
Moreover, the CDC technology is supported by multiple servers, including Microsoft’s Azure SQL Server and Oracle, making it the ideal solution for the movement of data.
How Does Change Data Capture Work?
Change data capture tracks changes in a source dataset and automatically transfers those changes to a target dataset.
Changes are synced instantly or near-instantly. In practice, CDC is often used to replicate data between databases in real-time. CDC instantly and automatically syncs databases as soon as the source data changes. Essentially, CDC eradicates the siloization of data.
Despite the introduction of CDC, most teams still use batch processing to sync data. With batch processing:
- data is not synced right away
- databases slow production to allocate resources for syncing
- data replication only occurs during specified “batch windows”
On the other hand, change data capture offers a new path forward. On a core level, change data capture:
- constantly tracks changes in a source database
- immediately updates the target database
- uses stream processing to ensure instant changes
With CDC, data sources include operational databases, applications, ERP mainframes, and other systems that record transactions or business occurrences. Targets include data lakes and data warehouses, including cloud-based platforms such as Google BigQuery, Snowflake, Amazon Redshift, and Microsoft Azure.
Once the data is replicated on the target database, teams can perform data analysis without taxing the production database.
In today’s 24/7 marketplace, this kind of setup is becoming closer to mandatory, as businesses cannot afford to slow production for any amount of time. Different technologies power change data capture offerings in today’s marketplace. These technologies include:
- Timestamps – Tracks “LAST_UPDATED” and “DATE_MODIFIED” columns. This method only retrieves changed rows, and requires significant CPU resources to scan all the tables.
- Table Differencing – Executes a diff to compare source and target tables. This will only load the data that differs. This method is more comprehensive than timestamps, but still places a big burden on the CPU.
- Triggers – Triggers are set off before or after commands that indicate a change. This produces a change log. With this method, each table in the source database requires a trigger, straining the system.
- Log-Based – Database logs are constantly scanned to detect changes. The changes are captured without adding additional SQL loads to the system. This removes significant stress on the CPU.
Change data capture enables teams to replicate data instantly and incrementally. CDC records data changes piece-by-piece, instead of relying on massive, all-at-once transfers.
This allows teams to stop treating data migrations as big “projects,” but rather as a byproduct of change data capture. With CDC, data is always up to date. The source database and target database are continuously synced. Bulk selecting is a thing of the past.
Only the modified data is synced with the cloud DWH. All other data remains static. This saves a tremendous amount of time, resources, and funding.
Change Data Capture Use Cases
CDC is a very flexible technology used across many niches, like data integration, data analytics, compliance, and so on. The technology’s capacity to track and capture data changes in real-time is what makes the CDC a practical (and beneficial) tool for businesses in the finance industry, insurance, transportation, healthcare, gaming, and many more.
You can find out more about why you need a CDC tool as well as the 10 best tools and their use cases.
For example, in retail and e-commerce, retailers can use the CDC to track inventory changes, update their item catalogs, and monitor sales and transactions, all in real time. Additionally, businesses in the e-commerce sector can utilize CDC to personalize recommendations and optimize their websites.
Another suitable industry for CDC use is manufacturing. Manufacturers rely on CDC tools to monitor the processes involved in production and ensure all relevant information flows smoothly between production systems and inventory management.
The social media and marketing industry also uses CDC tools. For instance, digital marketers and social media platforms use CDC to track customer interactions and content changes and optimize their marketing campaigns. With this powerful tool at hand, marketers can tailor customer-specific strategies that will boost the brand’s presence.
In the telecommunication industry, businesses use the CDC to manage network configurations and track call detail records. Additionally, businesses can optimize their network performance in real-time.
Change Data Capture in ETL (ETL CDC)
ETL, an acronym for Extract, Transform, Load, is a type of data pipeline that transforms extracted data before loading it to its target system, like a data warehouse or a data lake.
Data lakes are systems that contain a large amount of raw data without any clearly defined objective. On the other hand, a data warehouse contains filtered and structured data and has a specific purpose, mainly for BI (Business Intelligence) activities, most notably analytics.
With the help of ETL, a data warehouse stores massive amounts of data from various sources. But accuracy is paramount in this process as even the slightest undocumented change can influence outcomes. And this is where CDC comes in.
Before CDC technology, ETL could only extract data in bulk which slowed down the process and didn’t always provide accurate real-time information. However, CDC captures and delivers even the tiniest changes made to the data, step-by-step, in real-time.
For this reason, it brings many benefits to ETL pipelines. First, it simplifies and quickens the process, and second, it provides more reliable data in the system.
CDC can also work alongside ETL’s more modern counterpart – ELT (Extract, Load, Transform) – a more flexible process that doesn’t transform the data before loading it.
Change Data Capture Methods
One system can have one or multiple CDC designs. In addition, a CDC design can be implemented within the system – physically speaking – or externally on another computer system.
Not only that but there are many types of Change Data Capture methods, each suitable for different situations and data needs. Some prefer more intrusive methods, like creating database triggers to identify changes.
These triggers are procedural codes that automatically react to a certain operation in the database and activate once someone performs an insert, update, or remove operation in a database table. For example, it can activate once you add a new employee to a database table or increase their salary. The CDC will capture the change and deliver it to the system.
Others prefer less intrusive methods like following row timestamps and a transaction log to identify changes. In the first case, the CDC tracks the row’s metadata, specifically the modification dates, while in the second, it stores and reads the entire log to identify and deliver changes.
Log-based CDC methods and trigger-based CDC are the most common methods, but there are a few more examples worth mentioning.
- Polling-based CDC: This is a process that queries the data of the source system to identify changes. This is a suitable CDC method to be used when real-time data replication isn’t a prerogative and batch processing is entirely acceptable. However, polling can require some resources, especially when handling large systems.
- Timestamp or version columns: This is a CDC method best suited for data tables. Whenever a table row is updated, the timestamp or version column is automatically updated, too. CDC processes will periodically query data tables and pin-point changes by comparing versions or timestamps.
- Change tracking in database engines: Some contemporary database systems have built-in change-tracking mechanisms. One such example is Microsoft SQL Server. The feature records changes to tables, a suitable method for tracking and capturing changes within the database engine.
Importance of Change Data Capture
Data is the core of the modern economy. Businesses in every sector succeed or fail based on the data they collect, and what they do with that data. Today, companies in crowded markets gain a competitive edge not only from product differentiation but also from efficient data processes.
Key among these efficiencies is speed. In order to make the best decisions, and target the proper customers, businesses need to act on up-to-date data. According to Exasol’s 2019 Data Decisions Report, 57% of companies are negatively impacted by data access that is too slow or too poor in quality.
Companies must have the right data at the right time to compete in a 24/7 global economy. But many teams still rely on delayed batch processing to sync databases. Batch processing does not sync databases in real-time. And the batch method remains broadly popular.
Around 75% of business still rely on batch processing, but right now, across industries, a big shift is underway.
Many businesses are starting to use change data capture (CDC) to sync databases more efficiently. Change data capture empowers businesses to move at the speed of their data. CDC instantly and automatically syncs databases as soon as the source data changes.
Change data capture enables faster, more accurate business decisions while minimizing resource expenditure. The technology’s instantaneous data updates, cost-effective incremental changes, and light IT footprint offers a win-win-win to businesses. With the right CDC technology, companies can leave the inefficiencies of bulk processing behind, forever.
Change data capture empowers businesses to move at the speed of their data. Read on for an overview of what CDC is, and what it can do for your data operation.
Change Data Capture Best Practices
Businesses should follow some of the best practices to ensure their data is accurate, reliable, and performs well to effectively implement CDC. Some of the best practices in CDC include the following:
- Understand your data needs: Begin incorporating CDC by understanding your data integration requirements. Elaborate on data sources, targets, frequency of updates, and latency requirements. That will streamline the decision process and help you select the most suitable CDC method and architecture.
- Determine the right CDC method: Choose a CDC method that resonates with your requirements and specific use cases. Before settling on a method, consider factors like source system capabilities, data volume, and performance.
- Incorporate monitoring and logging processes: Ensure you have proper monitoring and logging mechanisms to track the quality and performance of the CDC tools. Setting up alerts for data anomalies and errors is also a good idea .
- Mind the scalability and performance capacities: Make sure your CDC architecture is robust enough to offer scalability and handle data as it grows. Businesses choose horizontal scaling options, load balancing, and optimizing query performance regarding massive datasets.
8 Benefits of Change Data Capture
1. CDC Generates More Revenue
Data is only as valuable as its relevance. A data point that records a customer entering a brick-and-mortar store is not very valuable 12 hours later. By then, the customer could have found dozens of other places to buy a product. This is just one example, among countless others, of how out-of-date data can botch revenue opportunities.
But businesses that use out-of-date data don’t just risk losing individual deals. Companies that consistently use old data open themselves up to long-term operational consequences. These risks are hard to measure up front, and they’re even harder to reverse once a business’s data infrastructure is built.
With change data capture, the risks associated with out-of-date data are entirely eliminated.
Change data capture provides teams with instant access to the most up-to-date data. This allows businesses to make decisions and take actions with the best data available. CDC necessarily improves the speed and accuracy of the data. Not only is data updated faster, it is also always 100% accurate.
Change data capture enables businesses to act on opportunities quicker. Companies can beat competitors to deals, all while cycling through a higher volume of opportunities. CDC also provides higher data quality for decision making. All of this empowers businesses to make faster, smarter decisions that generate more revenue.
2. CDC Creates Savings
90% of the world’s data was created in the last two years. The infrastructure of the internet, built in some cases decades ago, does not have the bandwidth to transfer massive volumes of data instantly.
This can become a serious problem for businesses that want to undertake projects with high data volumes, such as database migrations. These all-at-once data transfers severely congest network traffic, leading to cloud migrations that are slow and costly.
Change data capture, however, loads data incrementally as opposed to all at once. Each time a data point changes in the source system, it is updated in the target, requiring minuscule bandwidth.
With CDC, businesses are never subjected to large data transfers that crush network bandwidth. This reduces the cost of data transfers and saves weeks, months, and sometimes years of time.
3. CDC Eliminates Opportunity Costs
One of the core issues with batch processing is that the method inherently creates opportunity costs. During data transfers, batch loads slow down production databases and degrade performance. This can create opportunity costs in the form of lost deals.
Consider an e-commerce site with higher customer churn because the overtaxed production database slows down the site an hour each day. This is why batch processing requires specified “windows” when the production database is less taxed. But in a 24/7 global economy, there’s never an acceptable time to degrade the performance of a production database.
Change data capture, particularly the log-based type, never burdens a production data’s CPU. Log-based CDC capture changes directly from database logs, and does not add any additional SQL loads to the system.
Additionally, incremental loading ensures that data transfers have a negligible impact on database performance. What this means, in business terms, is that CDC eliminates the opportunity costs that arise when a business is forced to slow down vital tech infrastructure.
4. CDC Protects Business Assets
Data is not just something a company collects. In today’s environment, data is the lifeblood of a business. Data is a business asset just as much as equipment or property are.
However, mishaps that damage or delete data are common. For most businesses, such an event is not a possibility, but a probability. And for many companies, luck is the only thing keeping the incident from turning into a data catastrophe.
Change data capture protects data, a prime business asset, from deletion and destruction. By tracking changes not just to data, but to metadata as well, CDC offers companies that experience data loss a chance to repopulate impacted datasets.
Once data is gone, it can’t be regenerated. But with the protection of change data capture, businesses can recover their essential data to fuel further business growth.
5. Minimize the Strain on Operational Databases
If your business uses operational databases, it monitors the activities, employs analytics, and audits historical data. In this context, CDC helps narrow the margin for errors regarding performance. It creates a copy of the operational databases that are constantly updated and synched and is accessible to all users.
Since the traffic is pivoted toward the copies of operational data, the pressure on the operational databases is significantly lessened. This results in fewer database issues, eliminating the possibility of poor performance or downtime.
6. Reduce Issues with Incompatible Databases
Companies often face compatibility issues when connecting two or more databases. With CDC, businesses can boost the capacity to integrate with different software, which is more often incompatible with in-house databases.
CDC tools allow businesses of all sizes to become more versatile when choosing business applications without being limited by compatibility issues. In that context, designated teams within organizations can direct their focus on the business goals and not waste time dealing with incompatibility issues.
7. Better Data Security
One of the most significant benefits of CDC tools is to empower businesses to manage data accessibility with ease and accuracy. This particular capability translates to better data security.
With the right CDC tool, businesses can control the data flow based on how sensitive the information is. These practices enable businesses and teams to comply with various data protection laws in different countries.
Change Data Capture: Gaining the Competitive Edge
Change data capture is more than just a superior technology. For many forward-thinking businesses, CDC is a competitive advantage.
By staying several steps ahead of the market, companies with CDC can move at the speed of their data, and surpass the vast majority of businesses that are still stuck with batch processing.
Download our new eBook, The Business Case for Change Data Capture (CDC), to learn why implementing CDC is the best option for your business.
Simple Solutions for Complex Data Pipelines
Rivery's SaaS ELT platform provides a unified solution for data pipelines, workflow orchestration, and data operations. Some of Rivery's features and capabilities:- Completely Automated SaaS Platform: Get setup and start connecting data in the Rivery platform in just a few minutes with little to no maintenance required.
- 200+ Native Connectors: Instantly connect to applications, databases, file storage options, and data warehouses with our fully-managed and always up-to-date connectors, including BigQuery, Redshift, Shopify, Snowflake, Amazon S3, Firebolt, Databricks, Salesforce, MySQL, PostgreSQL, and Rest API to name just a few.
- Python Support: Have a data source that requires custom code? With Rivery’s native Python support, you can pull data from any system, no matter how complex the need.
- 1-Click Data Apps: With Rivery Kits, deploy complete, production-level workflow templates in minutes with data models, pipelines, transformations, table schemas, and orchestration logic already defined for you based on best practices.
- Data Development Lifecycle Support: Separate walled-off environments for each stage of your development, from dev and staging to production, making it easier to move fast without breaking things. Get version control, API, & CLI included.
- Solution-Led Support: Consistently rated the best support by G2, receive engineering-led assistance from Rivery to facilitate all your data needs.
Learn How CDC Boosts Business Performance
Minimize the firefighting. Maximize ROI on pipelines.
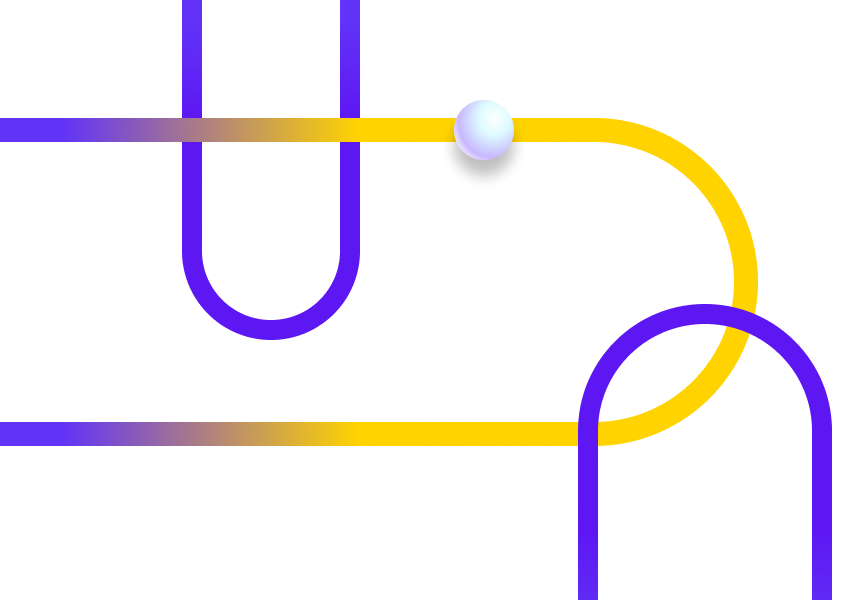
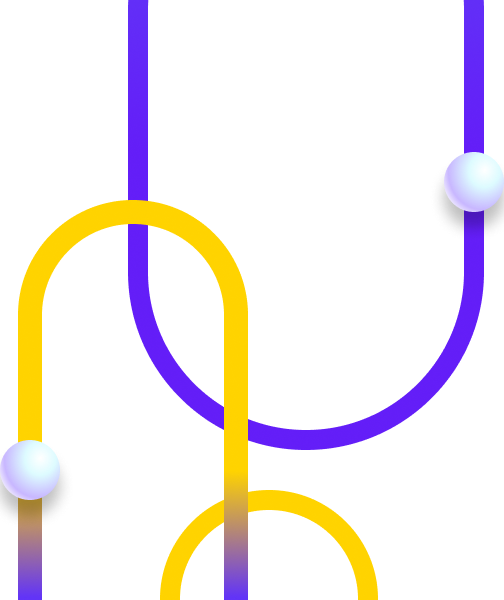