Up until recently, data warehouses were the go-to data storage and distribution technology for a majority of companies. For most companies, they’re more than enough to satisfy their data needs, but, for the few companies that receive increasingly more data each day, alternative methods are required; alternatives like Data Mesh.
Let’s analyze the differences between Data Mesh vs Data Warehouse and see what they’re about.
Introduction to Data Mesh and Data Warehouse
Data Mesh, a relatively new analytical data architecture was only created in 2019 by Zhamak Dehghani. This decentralized, domain-driven data organizational concept organizes data by domains. It catalyzes access to analytics and insights. On the other hand, the monolithic, centralized Data Warehouse technology stores data from different sources into a single architecture.
In short, the difference between data mesh vs data warehouse is how they are organized. Data mesh is decentralized, which allows for data to flow from different sources as required, while data warehouses centralize the data into one location.
What is Data Mesh?
The reason data mesh is considered revolutionary is because of the way it connects data sources by employing a decentralized system. This system is divided into 3 key components: source, infrastructure, and domains; all of which are governed by axiomatic principles.
The source can be any data center from which data can be requested. The infrastructure is the way by which data is shared between the source and the domains. The domains, on the other hand, are interconnected with each other allowing for data to be transferred through the whole system more efficiently.
This is the reason data mesh vs data warehouse is such a hot debate nowadays and why it’s such an important development. By decentralizing data sources, you almost completely eliminate the formation of bottlenecks, thus optimizing the entire process.
What is a Data Warehouse?
Data warehouse is a type of technology that has largely remained the same since its inception. In short, a data warehouse is a centralized database system that’s designed for storage, retrieval, analysis, and transfer of data.
Data warehouses act as a sort of repository for data in that sense, taking in data from multiple sources, processing it, and distributing it as required. This was traditionally seen as the best way to process data because of how efficient it was for structuring and systemizing data.
Similarities Between Data Mesh and Data Warehouse
The similarities between data mesh vs data warehouse can be found in their intended task. Both systems strive to store and process data in the most efficient way possible, the difference is how they go about this. The former does this by employing a decentralized model, while the latter uses a centralized model instead.
Data Mesh vs Data Warehouse: Key Differences
- Radically different data ownership: In a Data Mesh, different domains possess different data types. In a Data Warehouse, a data team external to the operational function is the owner, not the data producers.
- Opposing architectural approach: Data Warehouse follows a centralized approach, and Data Mesh follows a decentralized, domain-oriented approach.
- Varying data access: Data Mesh gives knowledgeable domain experts access to data. On the other hand, data warehouses and their consumers converse with the data repository, which might lead to delays or trouble.
- How they deal with complexity: Data Mesh is adequate for multifaceted data environments with a range of structures. Data Warehouses are suitable for structured and historical data.
- Scalability: Data Mesh treats data as products that can be independently scaled. Data Warehouses may need extra infrastructure scaling to handle large data.
Use Cases for Data Mesh
Traditional data warehouses suffer from a few key issues that data meshes workaround, chiefly, the problem of everything having to go through a central ETL pipeline and the problem of data transformation.
If you’re wondering what’s ultimately better between data mesh vs data warehouse, consider the following points. How often do you experience bottlenecks? How big is your data team, and how many data sources do you manage?
Data mesh is more beneficial if you have to manage multiple data sources, have a sizable data team, experience frequent bottlenecks from alternative methods, and have multiple data domains that require constant data.
Keep in mind that setting up a data mesh system is a much more complex task than setting up a data warehouse, thankfully, Rivery can help you with just that.
Use Cases for Data Warehouse
No matter how straightforward your company’s business model is, they can better themselves if they improve access to their data. Imagine a company that wastes hours on essentials like finding data or reporting daily. They will pay with lost productivity.
Investing in a Data Warehouse can minimize their downtime. Its data integration process enables all team members to access critical data. The same company that used to struggle to find relevant information will finally reach it. And, of course, there will be one team to control it. In this case, it’s evident that Data Warehouse is the winner in the Data Mesh vs Data Warehouse competition.
Collecting highly structured data from various sources gives workers a palette of information. It leads to business intelligence – the process of gaining valuable insights due to data analysis.
The main benefit of data warehouses is the level of data governance they afford you. With data warehouses, you have near complete governance over how your data is stored, and processed, leading to a more streamlined process in certain instances.
They’re great for helping you generate reports, access historical data, create visualizations, and are generally better for cybersecurity, and QA tasks, as well as achieving regulatory compliance.
Data warehouses are also quite scalable, however, that only applies up to a certain point. After a certain point, they cease being cost-effective solutions, necessitating other solutions instead.
Pros and Cons of Data Mesh
Up until now, we discussed the different characteristics of data mesh vs data warehouse, now let’s look at the specific pros and cons that the system has over the alternatives.
Pros
- Domain-oriented experts are responsible for their own data, allowing greater accountability and easier decision-making.
- Only a limited group of people owns the profound context around the data. For example, the sales domain deals exclusively with that domain-related topic.
- Facilitates scale and adjustable enough to suit the varying client needs.
- Domain leaders can easily access data and form valuable insights. By shaping data schemes and models, the team can further analyze domain-specific data, giving a sense of togetherness in the work setting.
Cons
- Implementing a decentralized architecture requires expert guidance and coordination. Storing data in various domain-oriented products is more strenuous than storing it in a straightforward, centralized Data Warehouse.
- The absence of a monolithic outlook on the dataset entangled analysis and reporting. Distributed approaches require a developed team to apply the data in the organization.
Pros and Cons of Data Warehouse
Now let’s take a look at the advantages and drawbacks of using a data warehouse.
Pros
- Made purposefully for reading large amounts of data and understanding connections and trends across them.
- Consistent data cleansing and transformation ensures they are constantly in a top-tier shape.
- This architecture has been present for centuries and never ceased to feel current.
Cons
- It isn’t made obvious who possesses and who takes charge of data produced by a domain. Consequently, the one-system-for-all approach lacks context.
- Data analysts are alienated from data production and its know-how producers, resulting in lost data value.
- If the analysts need to make slight alterations to the data, that could potentially take a lot of time. Furthermore, the produced data could be irrelevant or outdated.
Considerations for Choosing Between Data Mesh vs Data Warehouse
Before deciding on a data mesh vs. data warehouse architecture, a range of factors must be considered. Below, we’ll analyze them for you.
Business Requirements
Your unique business needs serve as a precursor of your future data architecture. You’ll never catch yourself off-guard by asking what your company intends to achieve. Check which stakeholders will interact with the data architecture. Ultimately, base your Data Mesh vs Data Warehouse decision on their counseling.
Data Complexity
To start collecting data, ask yourself what essential data cannot be excluded. Then, the extent of data volume and speed of production will be checked. Assess the types of data your company will be dealing with. It all comes down to what you are planning to achieve with the data-driven insights.
Scalability & Adaptability
The ideal choice will allow your company to deal with large masses of data smoothly without lost performance or workflow disruptions. The right data architecture for your company adapts to rapid market changes and effectively addresses customer needs, making keeping up with technology innovations easier than ever.
Data Mesh vs Data Warehouse: Conclusion
Data Mesh vs Data Warehouse notions may have contrasting outlooks on how they organize data, yet at some point, they meet, sharing a common goal. On top of their diversity is the modern decentralized versus the traditional centralized approach.
It is of utmost importance for organizations to measure their needs, perceived complexity, and core objectives. Only then will they make well-informed decisions that will serve their businesses. After all, Data Mesh vs Data Warehouse matters require data-led solutions.
Minimize the firefighting. Maximize ROI on pipelines.
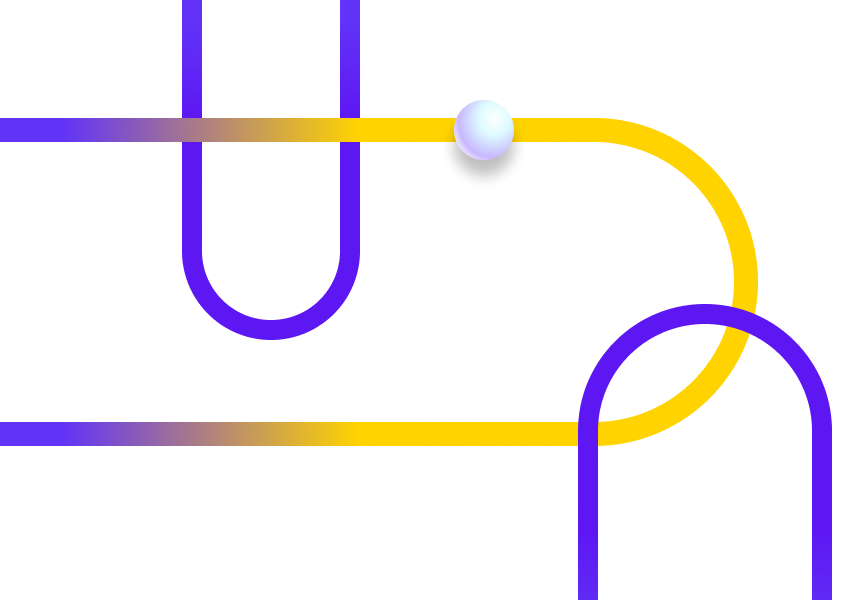
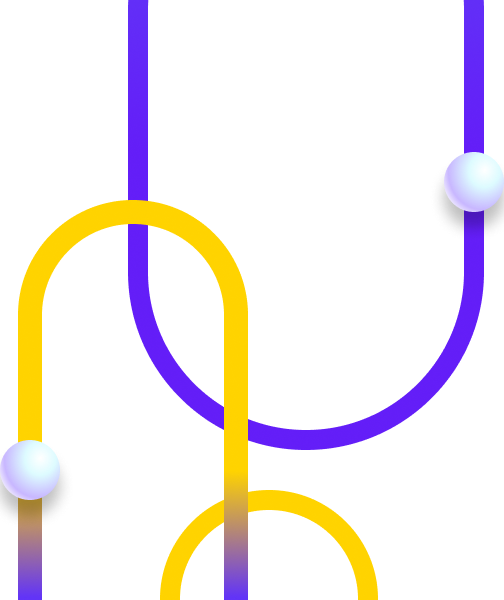