The average organization today juggles an astonishing 400 data sources.
Picture the sheer quantity of data distributed across apps, databases, spreadsheets, and various file types. Attempting to manage and decipher this data can often feel like trying to assemble a vast, perplexing puzzle with no clear starting point.
Enter data consolidation. By applying data consolidation techniques, you can turn the sprawling, tangled web of data sources into a structured, cohesive information pool ripe for analysis.
As data sources become increasingly complex and diverse and businesses scramble to manage hundreds of applications and databases, data consolidation becomes an essential tool in our toolbox. By reducing redundancies, enhancing data management efficiency, and facilitating seamless data analysis, data consolidation turns the intimidating puzzle into a clear, cohesive picture.
Data consolidation can help you quickly transform large and complex datasets into useful information. It simplifies data management, streamlines reporting processes, and provides an accurate view of your business’s data.
Let’s explore how data consolidation can help convert the inherent chaos of multiple data sources into actionable insights for your business.
What Is Data Consolidation?
Data consolidation is merging data from multiple sources, cleaning and verifying it by removing errors, and storing it in a single central location—such as a data warehouse or database.
This process allows you to access and manipulate different data types from one centralized point, turning raw data into actionable insights that drive better decision-making.
Here are 4 common examples use cases for consolidating data:
- Retail Company: A business in the retail sector integrates data from online sales, in-store purchases, and social media to optimize inventory and personalize marketing.
- Healthcare Provider: A healthcare business will consolidate patient records and lab results for accurate diagnoses and streamlined operations.
- Financial Institution: Many financial companies combine transaction data and customer profiles to enhance risk assessment and compliance.
- Manufacturing Company: Many manufacturing companies merge production data and supply chain metrics to improve efficiency and product quality.
Data consolidation also makes it much easier to access and analyze data across multiple sources. An average business has hundreds of applications and databases that must be managed, maintained, and kept up-to-date. As data sources become increasingly complex and diverse, data consolidation is becoming increasingly important.
For instance, imagine having customer contact information scattered across numerous spreadsheets within your system. Through data consolidation, you can bring all this data together into one comprehensive spreadsheet. In more complex scenarios, you might need to merge databases from different systems.
For example, imagine a retail company that has multiple brick-and-mortar stores across the country as well as an online shopping platform. As a result, the company’s customer purchase data, inventory data, and marketing data are scattered across several different systems. The data from the point-of-sale systems may be in a different format from the e-commerce data, and the marketing data may include additional customer engagement metrics.
Data consolidation comes into play by collecting data from all these diverse systems, transforming it into a common format, cleaning any inaccuracies or duplications, and then merging it into a single, unified database.
Now, with consolidated data, the company has a comprehensive view of its operations.
Data Consolidation Techniques
Data consolidation is a method of unifying data from different sources. There are five common techniques you can use to streamline data processes and consolidate data:
ETL (Extract, Transform, Load)
ETL is a traditional method for data consolidation. You will extract data from various sources, transform it into a suitable format, and then load it into a target system.
There are two main approaches:
- Custom coding: Custom scripts and programs are written to perform the ETL process. This method is flexible; however, it can be time-consuming and requires skilled developers.
- ETL tools: You can use specialized software tools to automate and manage the ETL process. These tools provide user-friendly interfaces, pre-built connectors, and strong data-handling capabilities.
ELT (Extract, Load, Transform)
ELT is a modern approach where you load data into a target system—often a data warehouse—and then transform it within that system. Therefore, you’ll use the power of the target system for transformations, making it suitable for large data sets and complex analytical queries.
Data Warehousing
Data warehousing consolidates data from multiple sources into a central repository. This enables efficient querying and reporting. Data warehouses are optimized for read-heavy operations and support complex analytical queries, making them superb for business intelligence and reporting.
Data Virtualization
Data virtualization gives real-time access to data without physically moving it. As a result, it creates a virtual layer that integrates data from various sources, which provides a unified view.
Moreover, this technique reduces the need for data replication and enables quick and flexible access to information.
Data Lake
A data lake is a storage repository that holds vast amounts of raw data in its native format. Unlike data warehouses, data lakes can store structured, semi-structured, and unstructured data.
Henceforth, this technique supports advanced analytics, machine learning, and big data processing, which provides flexibility for diverse data types and formats.
Additionally, these techniques offer distinct advantages based on the specific needs of your data strategy.
Data Consolidation Process
Data consolidation can seem overwhelming for beginners, but the process can be broken down into manageable steps. Here is a basic overview of the data consolidation process:
Identify Data Sources
Begin by identifying the various sources from which you need to consolidate data. These sources can include databases, spreadsheets, files, cloud applications, or external data feeds. For example, a retail business might have sales data in a CRM system, inventory data in an ERP system, and customer feedback in a survey platform.
Define Data Mapping
Once you have identified the data sources, you need to understand the structure and format of each source. Create a data mapping plan that outlines how the data elements from different sources will be mapped to a unified structure. For instance, mapping customer names, addresses, and contact details from different systems into a consolidated customer profile.
Extract and Transform Data
Extract the relevant data from each source according to the defined mapping plan. Transform the data as needed to ensure consistency, standardization, and compatibility across sources. This may involve data cleansing, data formatting, or data aggregation. For example, converting currency values to a common currency or standardizing date formats.
Merge and Integrate Data
Once the data is extracted and transformed, merge the datasets into a single consolidated dataset. Use techniques such as matching unique identifiers or creating standard data fields to align and integrate the data accurately. For example, merging sales data with customer data based on a unique customer ID to create a comprehensive sales report.
Cleanse and Validate Data
Cleanse the consolidated dataset by removing any duplicate records, resolving inconsistencies, and validating the data for accuracy and integrity. This step ensures that the consolidated data is reliable and free from errors. For example, removing duplicate customer records or resolving conflicting information.
Store in a Centralized Repository
Finally, store the consolidated data in a centralized repository such as a data warehouse or a unified database. This central repository becomes the single source of truth for accessing and analyzing the consolidated data. It provides a unified view of the organization’s data, simplifies data management, and supports efficient reporting and analysis.
Data Consolidation Techniques
To consolidate data from multiple sources, you may need to employ advanced data transformation techniques such as aggregation, joining, normalization, and filtering. These techniques are crucial for data cleaning, integration, and preparation.
- Aggregation: Combines multiple records from one or more sources into a single record by using functions such as sum, average, count, or maximum. For example, calculating the total sales for each customer from different data sources.
- Joining: Joins records from two or more sources based on a common field such as an ID or timestamp. For example, merging customer and order data based on customer IDs.
- Normalization: Transforms data from one format to another format using techniques such as encoding or scaling. For example, converting strings into categorical values (e.g., male/female) or scaling numerical values between 0-1.
- Filtering: Filters out unnecessary data from a source by using criteria such as date, time, or values. For example, filtering sales data between two dates.
These data consolidation techniques are crucial in data cleaning, integration, and preparation, key steps in the data management lifecycle, particularly in data analytics, data science, and business intelligence.
Importance of Data Consolidation
Data consolidation holds significant importance in modern business and technology landscapes.
Here are a five key benefits of data consolidation:
Improved Data Accuracy
Data scattered across multiple sources is prone to inconsistencies, redundancies, and errors. By consolidating data, organizations can standardize and cleanse the information, ensuring accuracy and consistency. Clean and accurate data forms the foundation for making informed decisions, conducting meaningful analyses, and gaining valuable insights.
Enhanced Data Quality
Data consolidation helps in improving the overall quality of data. By eliminating duplicates, resolving inconsistencies, and standardizing formats, organizations can ensure that the consolidated dataset is reliable, complete, and of high quality. This high-quality data drives better decision-making increases operational efficiency, and supports strategic initiatives.
Streamlined Data Management
Managing data from disparate sources is a complex and time-consuming task. Data consolidation simplifies data management by centralizing information into a single source. This centralized repository enables easier data access, reduces data silos, and facilitates efficient data updates, maintenance, and security measures. It eliminates the need to navigate through multiple systems, enhancing productivity and reducing administrative overhead.
Comprehensive Data Analysis
Data consolidation enables organizations to perform comprehensive analysis and reporting. When data is consolidated, it becomes easier to identify trends, patterns, and correlations across different datasets. Analysts and data scientists can access a unified dataset, saving time and effort in gathering and integrating data from multiple sources. This comprehensive view allows for more accurate and insightful data analysis, supporting strategic decision-making and driving business growth.
Data Integration and Interoperability
Organizations often rely on various applications, systems, and databases that operate independently. Data consolidation facilitates data integration and interoperability by bringing together information from diverse sources. It enables different systems to share and exchange data seamlessly, fostering collaboration, process automation, and the creation of integrated workflows.
Types of Data Consolidation
Data consolidation, the process of integrating data from disparate sources into a unified format, can take on different forms to meet the specific requirements of businesses and technology applications. Let’s dive into some prevalent types of data consolidation:
Type of Data Consolidation | Description | Use Case |
---|---|---|
Database Consolidation | Involves merging data from multiple databases into a single, unified database. Streamlines data management eliminates data silos and improves data accessibility. | Suitable for organizations with separate databases across different departments, regions, or applications. |
Application Consolidation | Focuses on integrating data from various software applications within an organization. Provides an inclusive view of business operations, customer engagement, and employee information. | Useful for companies using multiple business applications like CRM, ERP, and HRMS. |
File Consolidation | Unifies data stored in disparate file formats, such as spreadsheets, documents, and CSV files. Simplifies data access, enables multi-file analysis, and ensures data consistency. | Ideal for organizations dealing with data in various file formats and want to streamline data analysis. |
Financial Consolidation | Combines financial statements and other financial information from various business units into a comprehensive report. Provides an overall view of the organization’s financial performance and supports compliance, auditing, and financial planning. | For businesses with multiple subsidiaries or entities needing to maintain a unified financial report for auditing or financial planning. |
Data Warehouse Consolidation | Integrates data from various sources into a centralized warehouse. Supports complex queries, insightful reporting, and data-driven decision-making. | Perfect for organizations that require a unified and consistent view of data for advanced reporting and analysis. |
Cloud Data Consolidation | Merges data from different cloud platforms and services, such as SaaS applications and cloud storage systems. Centralizes data management, boosts data security, and streamlines data integration across cloud services. | Beneficial for organizations that leverage multiple cloud platforms and services and aim to enhance data security and management. |
The selection of a suitable data consolidation method depends on various factors such as the nature of the data, existing systems, business requirements, and scalability considerations. Often, organizations employ a mix of these methods to cater to their specific needs.
Data Consolidation Tools
Several software and tools facilitate the process of data consolidation. Each type of tool has its strengths and fits different use cases, depending on the specific needs of an organization and the nature and scale of the data consolidation task. Here are a few popular options:
Type of Data Consolidation Tool | Description | Best Use Cases |
---|---|---|
Spreadsheet Software | Allows for basic data consolidation tasks like merging files and standardizing data formats. | Best for small-scale tasks and simple data structures. |
ETL Tools | Designed to extract data from various sources, transform it into a unified structure, and load it into a data warehouse. | Handle complex data structures and large volumes of data; data warehousing projects. |
ELT Tools | Similar to ETL tools but with a different order: Extract, Load, Transform. They load raw data into a target and then perform transformations as necessary. | Ideal for modern, cloud-based data warehouses where transformation can occur after loading. |
Data Integration Tools | Combine data from different sources and provide a unified view. | Best used to merge data from different systems like CRM, ERP, and HRMS. |
Data Preparation Tools | Designed to clean, transform, and organize data before analysis. | Preparing data for data analysis and data science tasks. |
Data Validation Tools | Ensure that the consolidated data is accurate and reliable. | Used in scenarios where data accuracy is crucial, such as regulatory reporting. |
Data Visualization Tools | Represent the data in an easily understandable format like charts, graphs, or dashboards after consolidation. | Used post-consolidation for data exploration and to communicate insights. |
Data Warehousing Tools | Designed for managing large volumes of data and storing them in an organized manner. | Large-scale data storage and retrieval; enterprise-level data management. |
Cloud-Based Data Consolidation Tools | Enable the consolidation of data spread across different cloud platforms and services. | Used when organizations have data distributed across multiple cloud platforms. |
Data Consolidation in Finance
Data consolidation plays a critical role in various industries, particularly in finance. By merging data from multiple sources into one comprehensive view, financial institutions can significantly enhance their operations and decision-making processes:
- Unified Account Information: Financial institutions use data consolidation to merge account information from multiple sources, such as customer databases and internal systems of record. This results in an accurate and comprehensive view of a customer’s financial status.
- Portfolio Analysis: Data consolidation enables thorough analysis by merging data from different sources, providing a comprehensive view of investments across various accounts and time horizons. This comprehensive view facilitates informed decision-making based on the most current information.
- Fraud Detection and Risk Management: Data consolidation also contributes significantly to fraud detection and risk management. It helps financial institutions swiftly and accurately identify suspicious patterns or anomalies by consolidating data from multiple sources. This proactive approach aids in outpacing potential fraudsters and safeguarding customers’ funds.
Data Consolidation in Data Warehouse
Data consolidation in data warehouses involves gathering, integrating, and cleaning data from disparate sources and transforming it to align with the warehouse’s structure. This results in a data warehouse filled with standardized and clean data from various sources.
This data can be harnessed for business intelligence, reporting, and analysis. With a unified view of data, organizations can simplify their analysis and decision-making processes.
Data Consolidation and CRM Systems
Customer Relationship Management (CRM) systems often incorporate data consolidation capabilities. They provide a central repository for customer data, allowing organizations to consolidate customer information from various touchpoints, such as sales, marketing, and customer support. A consolidated ‘single source of truth’ enhances decision-making accuracy and keeps data organized, thereby minimizing the need for manual data management.
See Rivery’s solutions for CRM data management >>
Data Consolidation and Analysis
Data consolidation plays a vital role in data analysis and decision-making. By consolidating data from multiple sources, organizations can perform comprehensive analyses, identify patterns, uncover insights, and make informed business decisions.
Conclusion
Data consolidation not only simplifies data management and improves decision-making but also has the potential to uncover hidden insights and patterns that were previously obscured by fragmented data. By combining data from various sources, organizations may discover unexpected correlations, trends, or customer behaviors that can lead to innovative solutions, increased efficiency, and competitive advantages. This ability to extract valuable insights from consolidated data highlights the transformative power of data consolidation in driving business growth and fostering a data-driven culture.
Minimize the firefighting. Maximize ROI on pipelines.
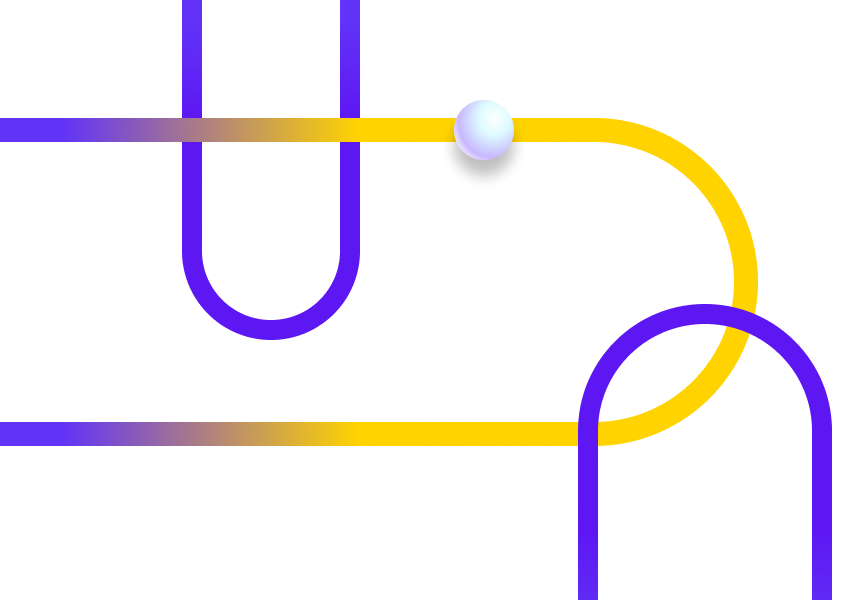
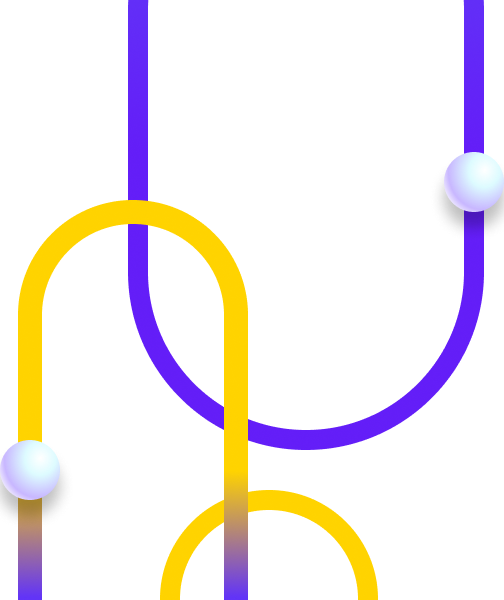