Companies today use data pipeline architectures to manage, report, and transfer data efficiently.
However, there are many aspects you should consider before choosing a particular data pipeline architecture or upgrading the existing one.
So what is data pipeline architecture? In this article, we’ll dive into the various data pipeline architecture, their fundamental parts and processes, and the crucial elements for their effectiveness. Keep on reading!
What Is Data Pipeline Architecture?
Data pipeline architecture is a set of processes and tools that makes the data transformation and transfer possible between the source system and the targeted data repository. It’s a comprehensive system that enables data routing, capturing, analyzing, and organizing.
Data pipeline architecture has three elements: a source, a transformation step, and the targeted destination. Generally, this system and tools are a piece of developers’ code that must work uninterruptedly.
To better understand the data pipeline architecture, we can divide it into 2 parts: a logical design and a platform design.
Logically, data pipeline architecture is the steps to transform and transfer the data from the source (a warehouse, application, or system) to the targeted repository (a cloud environment, data warehouse, or internal software). These steps usually include source data management, data preparation, transferring to targeted repository warehousing, and analytics.
On the other hand, the platform design of the data pipeline focuses on the tools you use. You’ll find applications, processes, storage, or data center migration platforms providing various data migration tools.
Key Elements Influencing the Effectiveness of a Data Pipeline
Data pipeline architecture best practices outline the importance of factors like time, costs, requirements compliance, and scalability on the effectiveness of the pipeline. These elements influence the effectiveness of data pipelines.
Cloud Storage May Not Always be Cost-effective
Even though we regularly praise cloud storage and cloud-based data migration, they can sometimes induce high costs. On paper, cloud storage services sound alluring and affordable.
But, businesses today operate with big data. That’s why, when designing your data pipeline architecture, you must always consider the cost-efficiency and choose the most productive architecture format for the particular platform.
Consider Compliance Requirements From the Beginning
When you build or implement your data pipeline architecture, you must consider the ever-changing business and regulatory requirements. The legislatures vary between states, so you need to make sure you follow the one where you’re based, especially concerning encryption and security.
Compliance with the requirements at the early stages is essential for avoiding legal matters, especially when using the data pipeline architecture to migrate Exchange Journal archives to cloud platforms.
Strategize for Performance and Scalability for Future Needs
Before you build and implement a particular type of data pipeline architecture, you must strategize for future needs and performance. One of the reasons for this is the constant increase and stratification of data.
Moreover, your high-quality data pipeline architecture should uninterruptedly distribute the data across multiple cloud platforms and clusters. That’s why organizations use container applications, multiple serves, and automation tools.
Data Pipeline Architecture: Why Do You Need?
Data pipeline architecture is the rudimentary system of data pipelines. As such, it’s responsible for taking, transforming, and moving data to a different system. Since most companies today have vast volumes of data flowing through them daily, having a streamlined data pipeline architecture can improve efficiency by allowing real-time access to said data.
Having this in mind, businesses and organizations need data pipeline architecture for more accessible and efficient data management, analysis, reporting, and transferring. Simply put, they allow productive data management.
Data Pipeline Architecture: Basic Parts and Processes
Every data pipeline architecture has several parts, such as a data source, processing steps, destination, standardization, corrections, etc. Below we’ll give you more details on the architecture’s essential parts and processes.
Data Source
The architecture integrates data from multiple sources. A data source is the primary server’s database from which the pipeline extracts, transforms, and transfers data.
Examples of data sources are SaaS applications, APIs, local files, or relational databases. The data ingestion pipeline architecture usually extracts the raw data from these sources through a push mechanism, webhooks, or API calls.
Extraction
The extraction is done through the “ingestion components” that enable reading the data from its data source. An example of an ingestion component is the application programming interfaces (APIs) in the data source. However, you must do data profiling before writing the API code for reading and extracting the data.
Joins
The data joins are also an essential part of the whole process. These are pieces of developers’ code defining the relationship between related tables, columns, or records in a relational data model. Joins are designed for extraction and transformation purposes.
Standardization
Standardization of processes, tools, and requirements is crucial for an effective data pipeline architecture. Each pipeline has its standardization criteria for efficiently extracting, transforming, transferring, monitoring, and analyzing data. Moreover, standardization is crucial for unifying your data in a standard format.
Correction
The data sources sometimes contain data with errors. Such data pipeline architecture examples would be the customer datasets with invalid or removed fields. In these cases, developers design the architecture to correct or remove this data through a standardized process.
Data Loading
After you extract, standardize, correct, and clean the data, it’s time to load it. Typically, we load the data in analytical systems, such as data warehouses, relational databases, or Hadoop big-data frameworks.
Automation
The automation process covers many aspects of the data pipeline architecture, and it’s usually used several times, whether continuous or on iterations. It detects data errors and clean-ups, gives status reports, etc.
Monitoring
Each data pipeline component—the hardware, the software, or the networking elements—can fail to operate. That’s why monitoring is essential; it can help you avoid functional problems at their early stages and assess performance.
Examples of Data Pipeline Architecture
The two data pipeline architecture examples are batch-based and streaming line data pipeline architecture. Below we’ll elaborate on both and show their differences and similarities.
Batch-Based Data Pipeline
Logically, a batch-based data pipeline means the data or records are extracted and managed as a group. It’s important to know that batch transferring isn’t “real-time” transferring because it needs time to read, extract, process, and transfer groups of data according to predetermined criteria by developers.
The batch-based data pipeline architecture works on a schedule or a timeframe that doesn’t recognize the new records, which is why it isn’t real-time. However, the batch-based data ingestion pipeline architecture is more popular than streaming data pipelines among companies with big data.
Streaming Data Pipeline
This one gives real-time analytics! The data often enters the pipeline in small sizes and flows uninterruptedly from multiple data sources to the desired destination. Along the way, the streaming data pipeline handles many pieces of data and their characteristics.
It’s standardized and automated to recognize errors, corruptions, breaches, invalid files, etc. On top of that, the streaming data pipeline usually connects to an analytics engine that enables organizations or users to analyze the data in real time.
Final Thoughts
Raw data can have elements that may not be important for your company. In these cases, using data pipeline architecture can help you manage critical information and simplify analytics. Data pipeline architecture boosts productivity, make important data readily accessible, and save time and costs for rechecks, clean-ups, and data errors.
If you want to simplify your data management and integration, turn to professionals. Get in touch with our experts at Rivery, and learn how to build your data pipelines!
FAQs
ETL (extract-transform-load) pipelines are a set of processes for extracting, transforming, and loading data in batches. On the other hand, data pipeline architectures don’t necessarily involve data transformation and can additionally provide real-time reporting and metrics, contrary to batch processing.
Organizations design data pipelines through a combination of analytics and software development. They go through 8 steps: determining the goal, choosing the data source, determining the data ingestion strategy, designing the plan, setting up storage, planning data workflow, implementing a monitoring and governing framework, and planning the consumption layer.
Yes, the ETLs are a subset of data pipeline architectures. Data pipelines are a broader concept.
An example of a data pipeline is the batch-based data pipeline which records, extracts, and transfers data groups from a particular data source.
Data flow refers only to the movement of data between the source and the targeted repository, while the data pipeline also considers transformation, analytics, and monitoring.
Minimize the firefighting. Maximize ROI on pipelines.
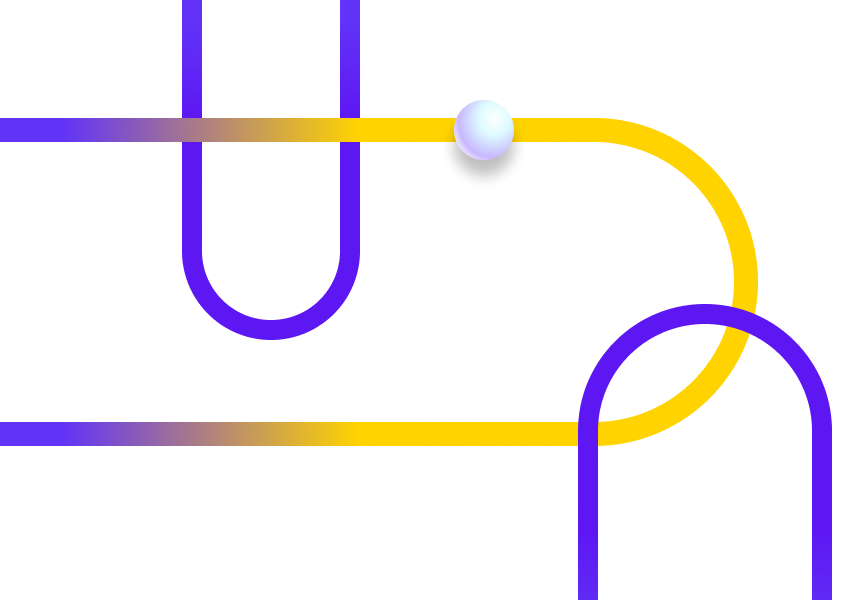
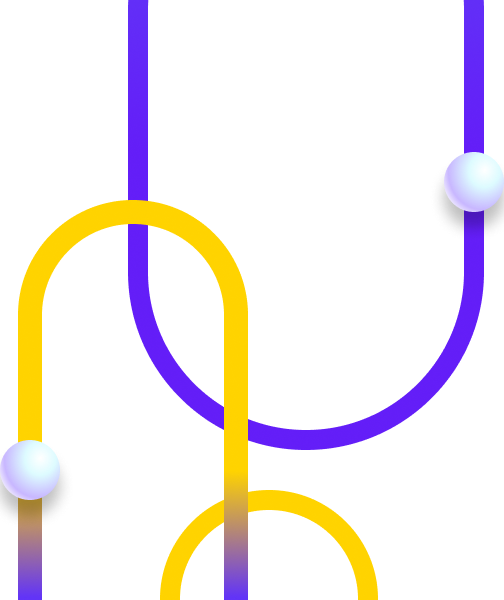