In a great article, the Chief Analytics Officer of Mode articulated the mind-boggling emergence of complex and fragile data stacks that doesn’t really align to how companies use data.
In his words, “because of the implicit technical divide (between technical and non-technical users) in the industry, data tools are almost always designed and sold for one audience or the other.”
Maybe it’s time to rethink the divide? Is there a new breed of analyst with the technical skills of a data engineer? The analytics profession is arguably at a crossroads, especially as technical and engineering skills are prioritized as part of data analysis roles.
Benn Stancil explains the effects of positioning analytics inside engineering, as “it suggests that great analysts need to be, first and foremost, great engineers. It also outlines a career path into analytics: Learn technical fundamentals, and then specialize.”
However, is this the right way to leverage the talent of a data analyst? He adds, “But this can be terribly wrong, as he argues that by and large, the hardest and most important problems analysts work on aren’t technical, or even mathematical.”
Maybe the evolution of the craft requires the newly created role of an “analytics engineer,” a middleman at the intersection between analytics and engineering. This would empower analysts to focus on what they do best – critical thinking.
“Rather than needing to be an impossible combination of statistician, developer, and business expert, data teams can hire creative historians, sociologists, and political scientists who are exceptional communicators rather than mathematicians who are passable coders.” When data analysts can focus on what they do best, analytics engineers could assist analysts with the technical skills needed.
The wrong old-fashioned approach for a modern data stack?
Tools, products and platforms catering to an ever-growing data stack are created to fit a role rather than a broader purpose within the DataOps ecosystem. Maybe, instead of focusing on building “code-free” tools for analysts without a technical background, the focus should be on removing friction between analyst and expert, not by replacing analysts with limited code-free solutions.
What’s more, when the segmentation of roles, responsibilities and skills becomes the foundation of which tools and systems are used, we run the risk of losing sight of data flows and overall DataOps ecosystem. Instead of being goal-oriented, teams can easily become tool oriented.
While specialization is a great thing, when this is driven by lots of bad tools your DataOps ecosystem might be in bad shape. Erik Bernhardsson explains the dangers of specialization in an overly-fragmented data team, often driven by the fact that tools are bad and hard to use. With so much fragmentation, besides losing track of the end goal, experts can also be a huge liability when biased towards picking tools that they have deep skills in.
What’s the way forward?
Instead of splitting by functionality, the data stack should be built based on how data is consumed. Stencil suggests that “the modern data stack doesn’t need a BI bucket and a data science bucket; it needs a unified consumption layer. To do our job well, we have to overcome the technical division, not be defined by it. Analytical needs don’t end at the code’s edge.”
There are lots of dangers when overcomplicating the data stack with too many tools and platforms. Orchestrating and syncing them is only part of the problem. Running and maintaining a data-stack which isn’t built with data usage and data flows at its core also means that they’re harder to manage and operate – with very few people getting a holistic view of how the data stack is run.
This multi-layered complexity, while built with simplicity and efficiency in mind, could end up resulting in the opposite – making data processes harder to understand, and insights harder to reach or manipulate autonomously by the broader organization.
Minimize the firefighting. Maximize ROI on pipelines.
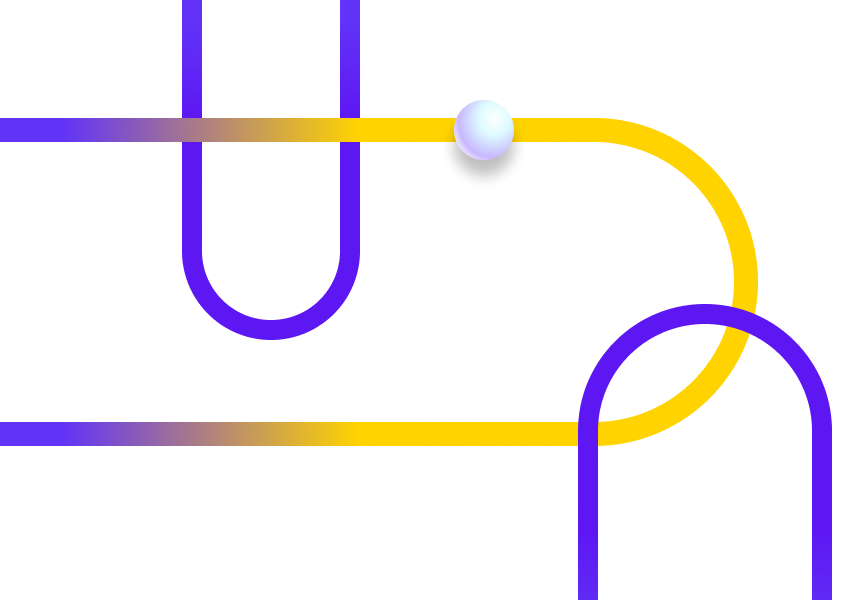
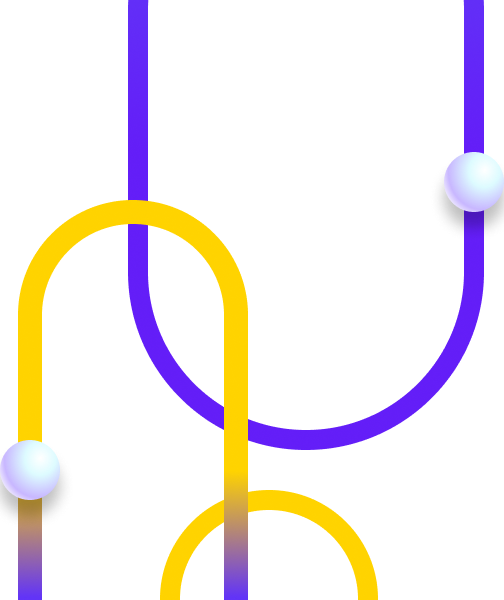