If you have not had the privilege of reading the book Touching the Void, I highly recommend it. It’s a story about climbers facing decisions and choices of unprecedented magnitude and consequences. Now you might be asking yourself, how does a story of survival relate to data?
Let me explain.
While creating coherent data warehouses doesn’t compare to the life or death situations described in the book, there are times when you need to make decisions quickly that you can’t back down from. You have to stand by and explain why a momentary decision creates a ripple effect of good and bad outcomes. It’s a touchy subject which can make or break data teams.
So when things don’t go as planned, it usually takes time and human resources to rectify any bad decisions. Creating a single source of truth to accomplish all your reporting needs is no easy task. It usually takes months to get your data in a state where you can accurately report on day-to-day activities. And then, adding new data sources or dealing with changes and source APIs can be detrimental to essential reports.
The Challenge of Interpreting DWH Data
The modern data stack attempts to alleviate the pain of creating this single source of truth. But instead of solving the problem, it just moves the data to your warehouse and you might find yourself facing the same challenges you had to start off with. A modern ELT platform allows you to deliver data into your warehouse of choice quickly. Some platforms even deal with source schema drift, and the good ones also allow you to transform the data once in the warehouse with push-down SQL.
The great ones even have Python transformation capabilities which extend the platform’s agility. Even with all of this managed data delivery, there is so much more to be done. Understanding the data once it’s in the data warehouse is not straightforward. It’s actually overwhelming. The problem of interpreting the data becomes your biggest pain. So in the end, all that you’ve accomplished is just having all of the data in one place.
This is when you realize the modern data stack did not solve the problem – it just helped you consolidate the data. This is what I call, “Touching the Void” moment. It’s the moment that you realize that all the work you’ve done so far has brought you to the realization that you can either give up now and accept your fate or rethink your stack to give yourself a chance of succeeding in the future.
How to Interpret DWH Data with Ease
To solve the problem you set out to solve, you need the right tools to help you interpret the data, and even more mechanisms to move data back to the source or activate the data to complete further actions. Most ELT providers know this and depend on customers adding additional tools to accommodate these requirements. At this point, decision-makers feel helpless and frustrated because they need to ask for more budget for the extra tooling.
Rivery was born from a BI consultancy that understood the modern data stack’s deficiencies and natively included tooling, allowing you to land the data, build your warehouse, gain insights, and subsequently take action on the data once it has landed in your warehouse.
Some of the keys areas of how Rivery customers are using the post-load tooling are:
- Data Warehouse as a source – Rivery can take data out of your warehouse and move it back to your sources to correct imperfections in data found in your single source of truth. Think of MDM use cases – Rivery allows you to correct anomalies found when various sources of data find discrepancies. This commonly fits into the category of Reverse ETL use cases.
- Activating or Actioning Data – Once your data has landed in your warehouse it can be monitored and used to action further transformations or even call external APIs to initiate further processing or even request data refresh to start the journey again. Our customers use this functionality to create ML use cases and interpret the results to begin extension use cases.
- Transforming DWH data using Rivery’s Python transformation capabilities – This in-built Python processing layer means that you have a fully extensible toolset to convert the data in any way you need. Recent use cases include PII Data Masking, Augmenting Raw Data with Open Source Insights, and Sentiment Analysis on DWH data.
If any toolset claims to cover all eventualities, then, of course, you should steer clear of that vendor, but when you have a platform born from the harsh realities of data warehouse design and real-world use – it’s worth exploring.
The Bottom Line
Running a data team oftentimes requires making difficult decisions that can set up teams for failure or success. To succeed in a modern data team you need a flexible approach and that comes in the form of a complete ELT platform. The ELT’s fully agile and customizable functionalities help to reduce risk, maximize resource efficiency, and minimize the costs of moving data as the sheer volume of data scales. All these benefits are what modern data teams crave and need to thrive in our fast-paced, competitive, and unpredictable economy.
Want to eliminate the risks of “Touching the Void” and speed up your data activation? Join the world’s top-performing data experts who use Rivery every day to push data into their data warehouse and back to its source to fix any silos and proactively transform their warehouse data in a few simple steps. Try Rivery for free, no credit card required.
Minimize the firefighting. Maximize ROI on pipelines.
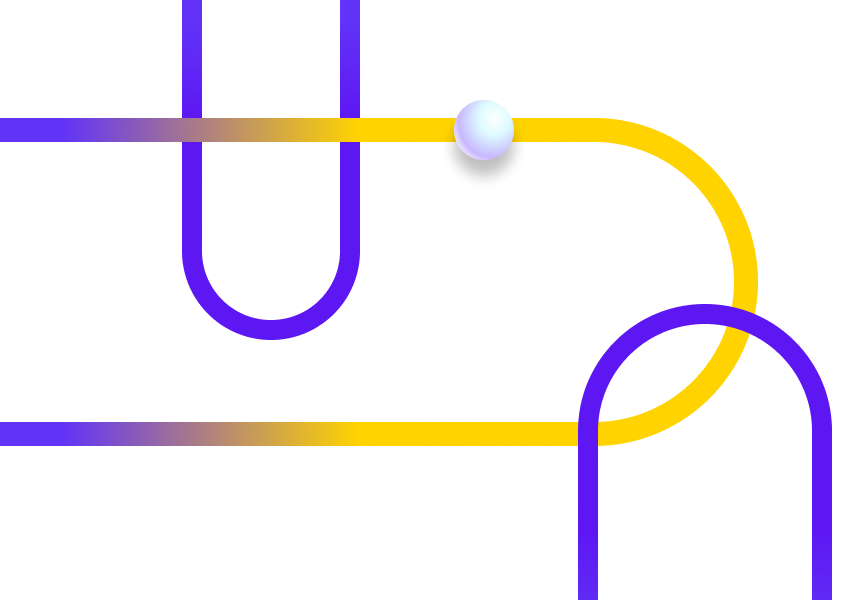
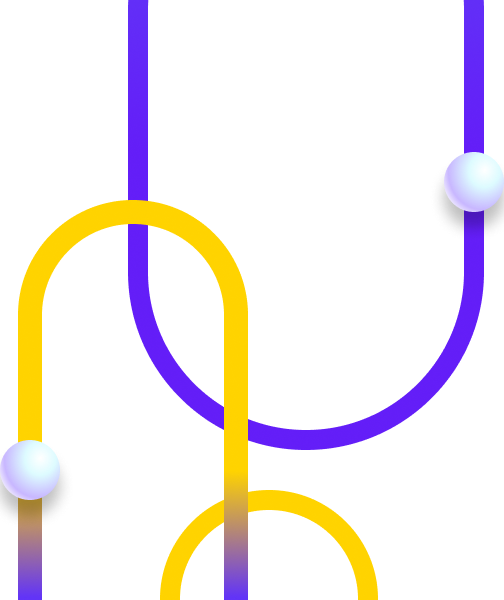