What is an Enterprise Data Warehouse?
EDW, short for enterprise data warehouse, is a central repository for storing information, more specifically, databases. It acts as a master database, keeping all other databases compiled by a business from various systems. Whether the data is structured, semi-structured, or completely unstructured, the EDW can consolidate it and make it coherent for further use.
The most common uses of an EDW include data analytics, data mining, and data comparison. This is done to get valuable insights into the business’s current operations and establish a strategy for future growth opportunities and innovations.
The Value of an Enterprise Data Warehouse
As mentioned, the primary purpose of data warehouses is storage. They can save large volumes of data from various departments, functions, physical recordings, and CRMs—all the historical business data you’ll ever need.
The second purpose is to turn data into actionable insights. Storing the company’s data in one place makes it easy to transform and analyze. Namely, in an EDW data warehouse, users can get a comprehensive overview of all data.
Moreover, they can quickly find specific datasets and discover how they correlate to the larger ones. This saves time and effort but also allows people with little to no tech expertise to gain access to complex data and be able to interpret it.
Understanding Enterprise Data Warehouse
When you want a comprehensive answer to what is an enterprise data warehouse, you need to look at the different components, concepts, and challenges associated with EDW. Below, you’ll find explanations for these aspects.
Key Concepts of an Enterprise Data Warehouse
The main concepts of an enterprise data warehouse EDW are as follows:
- Better Accessibility – The data warehouse makes complex sets of information more accessible to the end user. At the same time, they become easier to interpret and analyze. This leads to a more informed decision-making process, resulting in smarter business decisions.
- Data Structure – The main difference between a data lake and an EDW is structure. EDWs have a structured approach to data and even organize unstructured datasets to follow the same structure. They also allow for BI (Business intelligence) queries, which provide better insights.
- Non-Volatility – Unlike other databases, end-users cannot erase or overwrite data in an enterprise data warehouse, preserving historical data in its original form. Non-volatility ensures that this data is still accessible and can be used for analytical purposes.
Key Components of Enterprise Data Warehouse
There are four main components of an EDW:
- Data Sources – The original location of the databases, e.g., online systems, ERP, CRM, IoT devices, etc.
- Data Integration – By using a data integration approach like ETL (extract, transform, load), the EDW collects the data from the original sources and prepares it for further analysis.
- Data Storage – The final data model is loaded into the warehouse with added metadata storage.
- End-user Tools – A variety of tools and integrations that allow end-users to access the data and execute different operations with the data sets.
Common Enterprise Data Warehouse Challenges
The EDW data warehouse faces some challenges, too. The most common ones include:
- Time Consumption – Building an EDW takes a long time. It mainly depends on the amount of data, transformation and loading times, and establishing the necessary infrastructure. Implementing an EDW can take one to five years and requires careful planning, coordination, and resource allocation.
- Managing Data Quality – Since end-users can’t manipulate existing data, any errors, inaccuracies, or outdated information can go unnoticed and compromise the entire EDW.
- Complexity – Since data from multiple sources is stored in the warehouse, navigating it can become quite difficult. Dealing with large volumes of data and different formats without the proper modeling techniques can make the database nearly impossible to navigate.
The Benefits of an Enterprise Data Warehouse for Your Business
The shift from traditional data storage to enterprise data warehouse EDW seems justified, as there are many benefits to using an EDW.
Improving Data Quality and Consistency
EDWs store data in its original form, ensuring the information is consistent with the primary source. Additionally, the data can’t be altered or manipulated in any way, so end-users will be shown real historical data.
Enhancing Data Security and Privacy
Enterprise data warehouses implement various security measures, such as SSL encryption, that scramble the data and make it unreadable to anyone without access to the database. They also have built-in protection against data loss—either intentional or accidental.
Mitigating Risks and Ensuring Compliance
All end-users of the EDW have access to the same datasets and can audit the original sources, ensuring that no erroneous data is included in the EDW. Contemporary EDWs comply with the EU’s General Data Protection Regulation (GDPR) without additional checks.
Enabling Better Decision Making
When people have access to the entirety of a company’s data, they can make better and more informed decisions on the next course of action—whether that’s a marketing strategy, general management, or employee audits.
Boosting Operational Efficiency and Cost Savings
Granting all team members access to the data warehouse saves time. Instead of requesting a particular database from someone or struggling to interpret the data, users can access everything from the same place—already interpreted. This also cuts the cost of maintaining databases across several systems.
How to Implement an Enterprise Data Warehouse for Your Business
Once you understand the enterprise data warehouse definition, you can see why this is a great choice for your business. However, implementing an EDW is a process consisting of several stages.
Identifying Business Goals and Objectives
Whether you want to cut costs, optimize efficiency, take a data-driven approach to business, or simply improve the accessibility to data from multiple sources, think about the desired end goal and how an EDW can help you achieve it.
Building an Enterprise Data Warehouse Team
To build an EDW, you’ll need a team of experienced professionals from different areas—data architects, data analysts, and data management specialists, Business Intelligence experts, as well as a project manager to oversee everything.
Creating an Enterprise Data Warehouse Plan
The plan needs to specify exactly what the EDW will include and from which sources, how the loading and data transformation processes will be executed, and get the necessary resources for the execution of the project. This is also a good time to create a timeline and adhere to it.
Data Governance and Enterprise Data Warehouse Architecture
Management of policies and procedures for data management, in addition to security protocols, are all essential parts of data governance. Hence, these have to be established before the EDW is available to a wider audience. As for architecture, pick a three-tier model, which is currently the most popular approach.
Enterprise Data Warehouse Best Practices
Implementing an EDW data warehouse can be complicated, but you can ensure success if you rely on proven industry best practices.
Data Quality Control
EDWs allow you to conduct data profiling to validate the data contained in the database. During the validation process, you can resolve data issues or rectify any mistakes. You can hire a data auditor who will ensure data accuracy, find the proper documentation, and conduct regular audits.
Data Integration and Standardization
The data integration process combines information from multiple sources and transforms it into a uniform format that can be stored and analyzed by the EDW. During the integration process, all data is standardized to fit this format, i.e., all formats are converted to the one used by the EDW.
Data Security and Privacy Measures
Protecting sensitive data is a main concern. The best way to do so is by implementing access controls and user authentication mechanisms. In this way, only authorized personnel can access the database and make changes.
With SSL encryption, you can protect data not only in the EDW but also during transmission. Don’t forget about security audits and vulnerability assessments—they must be conducted regularly.
Data Analytics and Reporting
Once all data is standardized, users can conduct analyses and identify correlations within the data sets, as well as any trends or patterns. This can be done by integrating algorithms or analytical tools, and anyone—not only IT specialists—can conduct these checks. Based on the insights, users can create reports and take necessary actions.
Choosing the Right Enterprise Data Warehouse Tools and Technologies
When you look at what is produced by an enterprise data warehouse, you need to consider whether it fits in with your business needs. Choosing the right EDW tools and technologies requires doing the homework to find the right tool that will enable your organization’s growth and success.
Enterprise Data Warehouse Tools and Technologies
Choosing EDW tools and technologies will depend on factors like data volume and complexity, and analytical requirements. A few questions worth asking are:
- Is the EDW tool compatible with my data integration tool?
- Is the EDW tool compatible with my visualization tool?
Cloud technologies are notoriously popular due to their multifaceted storage capabilities., Cloud-based EDW platforms like Amazon Redshift, Snowflake, and Google BigQuery allow for more scalability, as well as faster and simpler integration.
Enterprise Data Warehouse Platforms and Solutions
EDW platforms have a wide range of features, so you’ll need to find one that meets the requirements of your business. Some choices include Google BigQuery, AWS or Snowflake. During the selection process, you need to consider security, integration options, scalability, and the overall performance of the EDW.
What’s the Right Enterprise Data Warehouse Tool for Your Business?
There’s no one correct answer to this question. You need to consider numerous factors—whether the data warehousing solution meets your business requirements, and whether there’s enough room for scalability and possibilities for integrations.
You should also check if the tool works with the technologies you are currently using and whether you can afford all the costs associated with operating and maintaining an EDW.
Final Thoughts
Implementing enterprise data warehousing can be a turning point in your business operations. By placing all data in one spot, an enterprise data warehouse can help you scale the right way and act on your data much faster. If you want to learn more about how to scale your data operations, check out how Rivery can help.
Minimize the firefighting. Maximize ROI on pipelines.
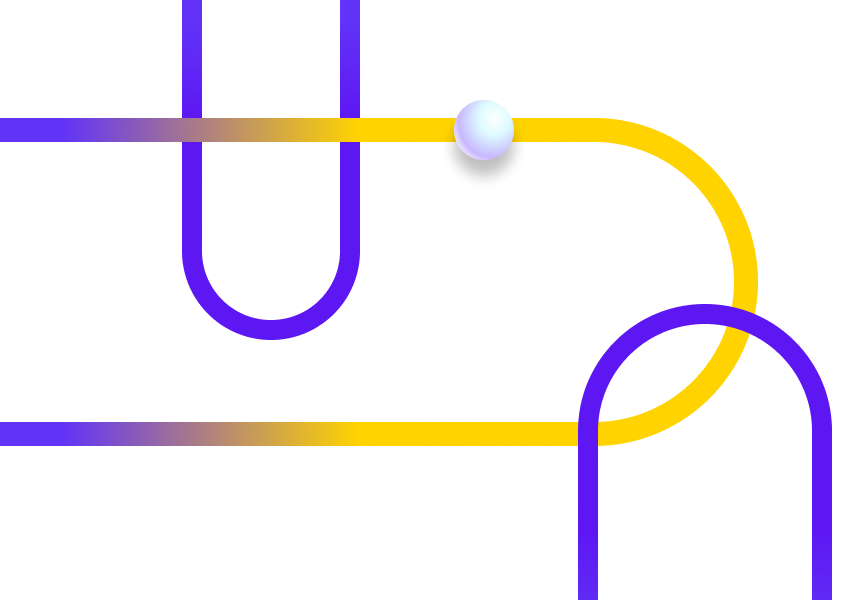
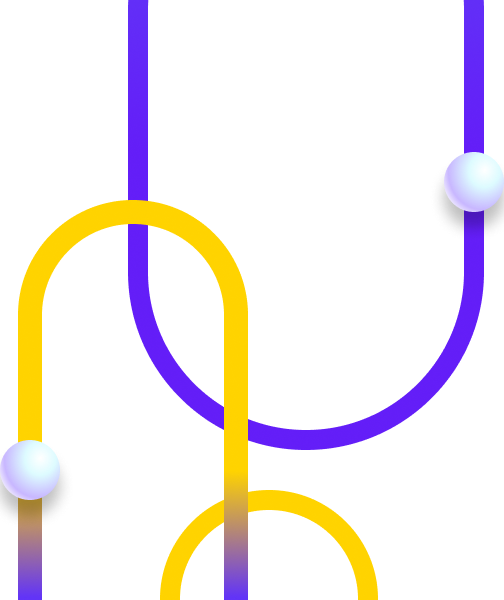