Data management is the process of collecting and organizing the data created by a company or organization. It also includes sorting, protecting, and managing that data so that a company can easily access it and use it for future decisions regarding the organization’s functioning. It can certainly help transcend the competition and create more opportunities. By sorting and managing your data appropriately, you get an accurate assessment of your entire operation and see how you can become more effective in planning and coordinating future endeavors.
That is why data management and data management tools are so important, especially going forward. There is a reason the plow is lauded as one of humanity’s greatest inventions, as it made the life of the average worker significantly easier. Data management is the plow of the 21st century, making your day-to-day operations all that easier.
It’s a powerful tool that helps businesses unlock the full potential of their data (both big and small) and maximize its value. In other words, it helps companies to stay on top of their game by giving them an edge over their competition.
Types of Data Management
Each company or organization is faced with its own unique set of challenges and requirements. As such, multiple types of data management have been created to satisfy those needs. Here are the most important types of data management you should know:
Data Quality
Data quality is, more often than not, an ongoing process that intends to instill a particular standard or quality in the data that your company works with. Setting a standard for data quality is essential for the following reasons:
- It helps ensure the accuracy of the data you work with
- It helps ensure the relevancy of the data you work with
Setting up a standard for data quality is especially important for companies that require fresh information to operate optimally. It’s also significant for companies that deal with a large influx of data daily, as it helps distinguish what data is relevant and what data is redundant and should be discarded.
Data Warehousing
Data warehousing is another type of data management that compiles data from one or more sources into a single source. The benefits of creating a data warehouse are that it makes data easier to locate for future reference. It also partially removes the requirement to scour for particular data in the future.
Data warehouses are particularly useful for research organizations or businesses that require detailed analytics to plan their future ventures. One of the main benefits of data warehouses is that they allow a historical overview of the data, allowing you to look at the changes that occur over time, which may help predict future trends
Key Components of Data Management
Data management is a complex subject that involves multiple dimensions. It encompasses storage, backup, analysis, transformation, integration, reporting, updates, synchronization, and security.
Data Architecture & Infrastructure
Data architecture refers to the high-level design or blueprint that directs how an organization structures and manages its data. It defines the standards, policies, and models that guide how data is collected, processed, stored, and utilized. Components of data architecture include:
- Data models and schemas
- Data governance policies
- Data integration frameworks
- Data storage and management strategies
This structure is manifested in the real world by data infrastructure, the actual hardware, software, networks, and other IT resources used to store, transport, and process data. Components of data infrastructure include:
- Databases and data warehouses
- Servers and storage systems
- Data pipelines
- Networking equipment
- Data processing tools
- Cloud computing resources
Think of data architecture as the “plan” for how an organization’s data should be handled, while data infrastructure is the real-world implementation of that plan; they work hand in hand to ensure the right data is available to the right people at the right time.
Data Quality Management & Data Cleansing
Poor data quality can lead to bad decisions, which could have disastrous consequences for any business. To ensure the accuracy and consistency of data, organizations utilize various processes to manage the quality of data throughout its lifecycle:
- Data cleansing
- Enrichment
- Profiling
- Validation
- Monitoring
- Auditing
Data Governance & Compliance
Data governance is the process of creating policies and procedures for managing data. It involves defining roles and responsibilities, setting rules for access and security, and ensuring that data quality standards are met.
Data governance deals with data encryption, access control, and compliance with privacy regulations such as GDPR, CCPA, and HIPAA.
Data Integration & Orchestration
Data integration is the process of merging data from multiple sources, providing a unified view of the business. This helps organizations gain a comprehensive picture of their data landscape, which can be used to drive better decision-making.
However, it’s easier said than done. The sheer variety of data sources, applications, and tools available today can be overwhelming. As the data volume grows and the number of data sources balloons out of control, the challenges of managing data sources effectively multiply. Efficient solutions that can help orchestrate all these disparate sources and tools are hard to come by.
Concepts like Extract, Load, Transform (ELT) & Extract Transform Load (ETL), Data Orchestration, Data Activation or Reverse ETL, and Data Operations come into play. These, while essential, add further layers of complexity to an already demanding environment a business has to operate in.
Data Visualization and Analysis
Data visualization translates PPP mm data into intuitive visuals. These could take multiple forms: charts, maps, or graphs – all of which can help uncover trends and patterns that might otherwise be missed. Since the human brain can interpret visual information more quickly and efficiently than raw data, effective data visualizations can greatly improve decision-making processes. Popular data visualization tools include Tableau, Qlik, and Power BI.
Data analysis involves using sophisticated algorithms to mine data, uncover hidden patterns, and generate valuable insights. It involves various statistical, mathematical, and programming techniques. Data analysis can be descriptive (what happened), diagnostic (why it happened), predictive (what might happen), or prescriptive (what action should be taken). The insights derived from analysis are then used to identify problems and opportunities and help organizations make informed decisions.
Benefits of Effective Data Management
By leveraging the right tools, processes, and techniques to manage their data effectively, organizations can drive better decision-making, optimize operations, and uncover hidden opportunities. Ultimately, effective data management is essential for any business that wants to remain competitive in the ever-changing digital landscape.
- Better Decision-Making: Tools for data visualization, reporting, and business intelligence can turn raw data into actionable insights. This empowers businesses to make well-informed, data-driven decisions.
- Enhanced Data Quality and Consistency: Data quality is of strategic importance for any business. Poor data quality leads to inaccurate insights, wasted resources, and increased operational costs. By implementing a robust data management strategy, organizations can ensure that their data is accurate and up-to-date.
- Improved Data Security: Nothing derails a business or organization faster than a data security breach. By implementing a robust data management strategy, organizations can ensure their data is secure and compliant with all relevant regulations. This helps to reduce the risk of costly fines and any potential reputational damage associated with a data breach.
Bringing Effective Data Management Strategies to Life
To bring an efficient data management strategy to life, it’s crucial for companies to carefully pick the right tools, approaches, and methods. The aim is to create an efficient, automated system for managing data from end to end.
- Set Clear Data Management Goals: To win at data management, it’s essential to lay down clear goals that sync with business objectives. These could vary from enhancing data quality to fulfilling legal requirements or trimming operational costs.
- Create a Data Management Plan: A comprehensive data management plan outlines the strategy for handling an organization’s data throughout its lifecycle. It addresses key aspects such as data governance, data documentation, data quality procedures, and data lifecycle management.
- Select the Right Tools and Techniques: Companies need to handpick the correct tools, methods, and procedures to reach their data management goals. It’s vital for organizations to take a moment to assess different alternatives and select the ones that serve their needs best.
- Build Your Data Management Dream Team: Creating an efficient data management team is crucial for successful data management. This team should comprise individuals with diverse skills, including data analysis, data governance, data security, and more, all working collaboratively towards a common goal.
- Ensure Data Governance and Compliance: Set data privacy, data retention, and data access policies that are in line with data governance frameworks relevant to your business and industry.
Challenges of Data Management
The mentioned data management strategies can help you overcome the numerous challenges of data management, which require proper addressing. A proper data management plan and clear goals can help you overcome large volumes of data, which is one of the most significant challenges.
A synergetic data management team that uses the right data governance tools can eliminate the many redundancies of data management. By setting up automatic processes, you streamline the entire data management process.
Consolidation is another serious challenge, which only becomes more challenging as you add more data sources. The proper tools can also help you overcome this issue by centralizing your multi-source data into one data silo or data warehouse, thus making it a lot more accessible than before.
However, of all the data challenges, data security is one of the most concerning and requires a many-sided approach to resolve appropriately. Developing the proper data security habits is instrumental, as there, sadly, are no data management tools that can help after one makes a poor choice. However, various data security tools can increase the safety of your data transfer processes.
Data quality is likewise a tricky challenge, which only gets more complicated as more data enters your system. However, that challenge can be easily overcome with the right data governance tools, regular audits, and proper management and sorting tools.
Best Practices for Effective Data Management
Data Quality Control & Cleansing
High-quality data is the backbone of any successful business. It’s crucial to employ best practices throughout the data lifecycle to maintain the integrity, consistency, and accuracy of your data. Here’s how you can achieve this:
- Define Clear Standards: Set clear, specific standards for data entry to ensure consistency. This includes predefined formats for dates, addresses, and other essential fields.
- Clean Up Your Data: Utilize techniques like data profiling, data cleansing, and data enrichment to ensure high-quality data.
- Audit Regularly: Carry out periodic audits to identify errors or inconsistencies in your data. This helps in maintaining data accuracy and timely correction of any inaccuracies.
- Implement a Data Governance Framework: Setting rules and procedures for data handling will help ensure data is maintained appropriately throughout its lifecycle.
Data Integration and Interoperability
To ensure smooth data flow across various systems, businesses must focus on data integration and interoperability. Without this crucial step, data silos will form, leading to inaccurate insights and missed opportunities.
Here are some ways you can achieve that:
- Use Data Integration Tools: Employ data integration tools that can pull data from different sources and load it into the target destination – a data lake or data warehouse, consolidating all that data into a single, unified view. These tools can automate data pipelines and the entire data process, ensuring data is seamlessly integrated at scale and speed, reducing the risk of errors.
- Establish Common Standards: Establishing common data standards across your organization can aid in data interoperability. It ensures that data from various sources can interact with each other effectively, allowing for more seamless integration.
- Implement APIs: APIs can help bridge different systems, allowing them to communicate and share data more efficiently.
- Data Mapping: This involves linking data from one system to similar data in another system. By understanding how data is related, you can integrate data across multiple systems more effectively.
- Data Transformation: Convert data into a common format with SQL or Python to answer complex use cases and enable it to be accessed and used across multiple systems. This is particularly crucial when dealing with legacy systems that may not use the same data formats as modern applications.
Data Security & Privacy
In the digital age, the need for robust data security and privacy measures can’t be overstated. It’s crucial to employ strategies that safeguard data from breaches and unauthorized access. Here are some top best practices:
- Data Encryption: Encrypt data, both at rest and in transit, rendering the data unreadable to unauthorized users.
- Implement Access Controls: Limit data access to authorized personnel only. Role-based access controls can ensure that employees can access only the data that is necessary for their role.
- Use Data Anonymization Techniques: Remove personally identifiable information from data sets, ensuring that individuals cannot be identified from the data.
- Regular Security Training: Educate your employees about the importance of data security, potential threats, and best practices to protect sensitive data.
Data Governance & Documentation
Data governance plays a crucial role in establishing policies and procedures that regulate data management. It also ensures consistency, accuracy, and security of data. Here are some best practices:
- Establish a Data Governance Framework: Set clear guidelines about data collection, storage, management, and usage.
- Assign Data Stewards: Data stewards are responsible for maintaining data quality, implementing governance policies, and ensuring compliance with these policies.
- Develop a Data Catalog: A data catalog serves as a single source of truth about all the data in the organization. It can help in understanding the data’s origin, usage, and relation to other data elements.
- Regular Audits and Reviews: Regularly review your data governance practices to identify areas of improvement.
Choosing the Right Data Management Tools and Technologies
Data Management Tools and Solutions Overview
Data stacks include a combination of tools and technologies that enable efficient data management. Here is an example of what a data stack in an enterprise-level organization might look like:
- Data Sources: These include various types of databases (relational, NoSQL), operational systems (like CRM and ERP), and external data sources (third-party APIs, IoT devices, web scraping).
- Data Warehouses: This serves as a central repository of integrated data. Snowflake, Amazon Redshift, Google BigQuery, or Azure Synapse Analytics could be employed here.
- Data Lakes: For storing raw, unprocessed data, organizations might use solutions such as Amazon S3, Azure Data Lake Storage, or Databricks
- Data Virtualization Tools: Tools like Denodo or Cisco Data Virtualization might be used to provide an abstract layer that allows unified access to data across disparate sources.
- Enterprise Service Bus (ESB): MuleSoft Anypoint Platform or IBM Integration Bus could be used to ensure effective communication between different software applications within a service-oriented architecture (SOA).
- Data Catalog Tools: Alation or Collibra might be used to maintain a metadata repository for easier discovery and management of data assets.
- Data Governance Tools: Solutions like Informatica Axon or IBM Watson Knowledge Catalog could help establish protocols for data quality, privacy, and compliance.
- Business Intelligence (BI) Tools: Finally, tools like Tableau or Power BI would be used to analyze the data and generate insights for decision-making.
- Data orchestration: Data orchestration refers to the automated organization, coordination, and management of complex data processes. Tools like Apache Airflow, Google Cloud Composer, and AWS Step Functions provide a platform to design, schedule, and monitor data pipelines with features for error handling, workflow scheduling, and alerting. These tools play a critical role in optimizing the overall data architecture, ensuring that the right data is available to the right tools at the right time.
- Data Integration Tools & Platforms (ETL/ELT):
- ETL involves extracting data from various sources, transforming it (e.g., cleaning, formatting) to make it suitable for analysis, and then loading it into a data warehouse. This approach is suitable when you have predefined requirements for data transformation.
- ELT, on the other hand, involves extracting data, loading it directly into a data lake or warehouse, and then transforming it as required for analysis. This approach is more flexible and scalable, making it suitable for big data applications.
- The hallmark of superior data integration tools lies in their dynamic flexibility and capacity to go beyond standard extract, load, and transform. Modern ELT tools support both custom and no-code development, facilitating the creation of intricate, end-to-end ELT pipelines regardless of use case. Tools like Rivery, a SaaS ELT platform, let you move your data with ease without setbacks and manage your DataOps from start to finish.
This is a broad structure of a typical data management stack, which can be tailored according to the specific needs of the business. Some organizations might incorporate AI/ML platforms, real-time analytics tools, data operations (DataOps) tools, or Master Data Management (MDM) tools into their workflows.
The Future of Data Management
As Big Data continues to expand, so does the complexity of our data stacks. We are dealing with more data sources, formats, and platforms than ever before, which significantly complicates the data management task in front of us.
The future of data management is not about simply adding more shiny tools to our toolbox. It’s about how to effectively integrate our existing tools to create end-to-end workflows, from the raw data all the way through to the insights we derive from it.
What’s next for data management? It’s all about building continuous data pipelines to help organizations squeeze every drop of value from their data. How do you achieve that hefty goal? Well, it all starts with selecting the right data integration tool, we hope this article helps.
Minimize the firefighting. Maximize ROI on pipelines.
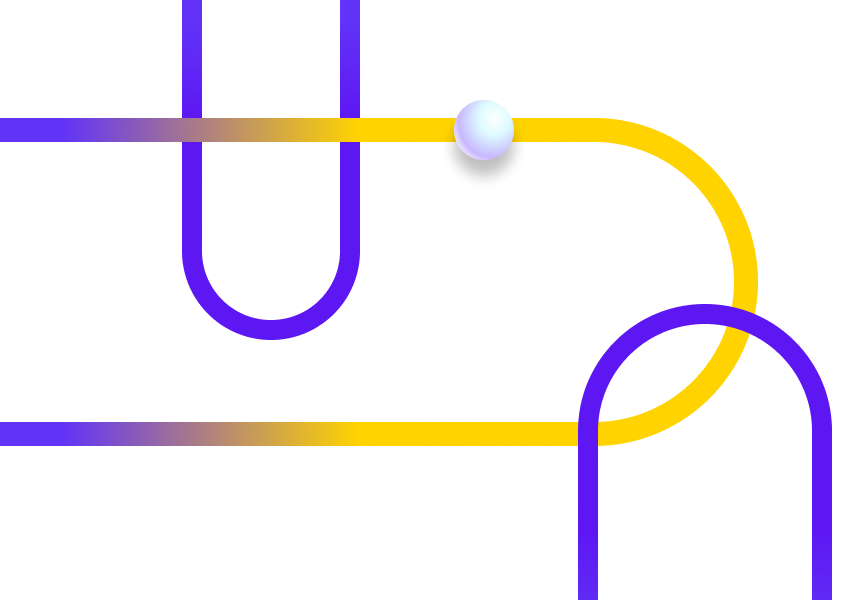
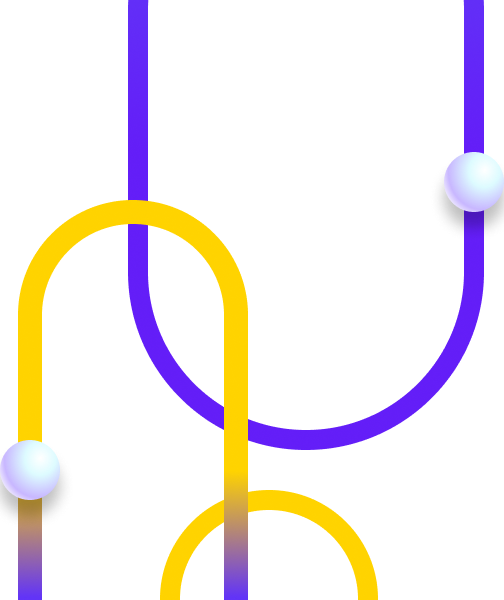