In the fast-paced corporate world of today, everything is levied on modern data: data on sales, data on growth, data on performance, and so on. Regardless of the size of the organization, business entities should not expect to reach exponential growth if they don’t harvest proper data to influence business decisions.
Businesses are basically required to collect pools of customer data to be able to sustain their business goals. This yields fertile ground for data multiplication of applications running on data in the vast realm of technology.
Recent stats show data generation is foreseen to expand more than tenfold, leaping from 33 ZB (zettabytes) in 2018 to a massive 175 ZB by 2025. With such an overwhelming amount of data at hand, errors are bound to happen if data isn’t properly harvested, hence, data orchestration.
What Is Data Orchestration?
Data Orchestration is the process of harvesting big data from a variety of data-storing locations for the purpose of combining and organizing it. Furthermore, it makes data available for data analytics tools, which are programs and software that collect and analyze business, customer, and/or competitor data.
The main purpose of data orchestration is to allow data analysis tools to filter, sort, organize, and publish complex data within a data-storing cloud. Actions like monitoring, composing, and lining up data pipelines using data from different sources are what data management solutions offer.
Why Enterprises Need Data Orchestration
First and foremost, data orchestration allows businesses to computerize and facilitate decisions based on data analysis. It will connect the business’s storage outlets into a single pool so that data analysis tools can easily access and analyze heaps of data whenever needed.
Enterprises that practice intricate data management have uninterrupted access to both existing and incoming data, making it easier for data analysis tools to process information faster. Big data orchestration makes decision-making more efficient and accurate.
Benefits of Data Orchestration
As mundane as it sounds, data orchestration can boost the efficiency levels of your organization. Yet, there are more layers to the advantages of this part of data management, like reducing costs, being compliant with data privacy laws, and more.
Reduced Costs
Cost reduction is probably the most appealing trait of data orchestration. Naturally, all companies aim to minimize their costs while expanding their profits. To do that, companies need to harvest substantial amounts of data from different outlets, which is not an easy task.
The IT experts within your company can lose hours extracting, organizing, and categorizing data for data analysis tools to process the information. Other than being a strenuous task, if done manually, it can cause large error margins, which is why companies prefer to stay on the safe side and practice data management.
Besides shrinking the window for errors, data management will ultimately help your business reduce the compensation bill. Moreover, you won’t have to hire more staff to perform data analysis – the software will carry out the task.
Eliminated Data Bottlenecks
Data bottlenecks are the stumbling blocks preventing data flow from coursing through filters and rendering accurate information. Mainly, bottlenecks appear as the result of mishandled data or lack of data-handling capacities, especially in heavy data traffic.
Using data orchestration enables your business to automate the data sorting process while also prepping and organizing data. This leads to less time spent on data harvesting and preparation.
Ensured Data Governance
Adhering to data governance standards is an important practice for enterprises, which is another point where data orchestration can help. Data management refers to the process of regulating the data used in corporate organizations by using standards and policies.
If your data comes from several different outlets, keeping data flow in check and monitoring the proper processing of data becomes challenging. Now, by using data orchestration tools, your company’s governance plan can easily connect all outlets while simultaneously complying with your data-processing strategy.
Challenges/Limitations of Cloud Data Warehouse
Storing your enterprise’s data in a cloud warehouse is more than essential for the success of your business. Namely, the warehouse is the main depository for external and internal corporate data sources.
The main use of a data warehouse is to back strategic business decisions by providing data analysis. However, there are a few limitations to using data warehouses, like:
- Non-unified cost scheme;
- Lack of skill options;
- Extended cloud security and governance;
- Movement of data;
- Missing standardization.
Data Orchestration Tools/Platforms
Tools or data orchestration platforms for data management allow businesses to automate their data-driven processes. Strategic and targeted audience decision-making are some of the crucial actions a business can take to accelerate revenue or reach a broader audience.
By using data orchestration tools or platforms, all of your data will be harvested, categorized, merged, when and if needed, and prepared for analysis. Moreover, data orchestration platforms are suitable for machine learning, too.
Rivery Data Orchestration
No-Code Solution
Centralized DataOps
Automated Flow
Compatible Data Transformation with Top Cloud DWHs
Implementation of DAGs;
Python-based tool;
Incorporates several data orchestration projects.
No coding requirements;
Data management during flow;
Error-handling features.
Open-source;
Free to use;
Able to integrate with the Google Cloud Platform, AWS, Microsoft, etc.
Lightweight;
Open-source;
Ready-made automated setup.
Data Orchestration Process/Framework
The term orchestration framework (OF) refers to a tool that computerizes the data orchestration process. Nowadays, many artificial intelligence programs use data orchestration to deliver clean data.
A configurable framework governed by business rules, the orchestration framework is used to determine the business scenarios in question. It also automates logistics processes and unifies data integration.
Data Orchestration vs. Data Visualization
Data visualization refers to the process of visually presenting information using graphics, highlighting schemes, and following data trends. All of the actions help the user gain insight at a faster pace.
Rather than opposing data orchestration, data visualization can help data analysis tools understand different data patterns. Ultimately, such actions will make the data analysis process easier.
Data Orchestration vs. ETL
In IT, ETL revolves around a three-step process for extracting, transforming, and loading data into a data output container. ETL and data orchestration complement each other to deliver spotless data to the end user.
Data Orchestration vs. Data Pipeline
Data pipelines carry massive amounts of data that’s transferred from one data outlet to another. Data management wraps up the work of data pipelines by extracting information and preparing it for the data orchestrating tools to process and segment data properly.
Conclusion
Practicing data orchestration for your enterprise can be the most cost-efficient business transaction you make. Furthermore, it helps you make more accurate and result-driven business decisions.
Managing and orchestrating your data can be complex and can really hinder your work if not done right. If you want to stop working for your data and make it work for you, reach out to Rivery and let’s see how we can solve your data issues.
FAQs
Orchestration in IT refers to the process of harvesting real-time data from multiple data sources and integrating them into a single pool for easy access and management of collected data.
Data pipeline orchestration means moving and combining different data from multiple sources to prepare data for analysis and reach the end-user stage.
ETL stands for Extract, Transform, and Load data, so an ETL orchestration is a mechanism that constructs large data pipelines.
Basically, cloud orchestration is an approach in data analysis that automates the tasks incorporated into managing the connections and operations of data workloads on internal and external clouds.
Automation refers to computerizing a sole process, or a minuscule number of corresponding tasks, whereas orchestration deals with managing several automated tasks.
An AWS (Amazon Web Services) orchestrator is assigned with the automation of management, coordination, and institutionalizing of elaborate computer systems, middleware, and services.
Orchestration of data is possible by using data orchestrating tools and platforms such as Apache Airflow, Metaflow, and others.
An orchestration service is possible to create by identifying the problem and the solution, identifying the data needed for orchestration, determining the rules for orchestration, specifying the cross-reference information for the orchestration, and identifying the service request for orchestration.
Container orchestration helps with the redistribution of a single application across various surroundings without needing to reassign the task.
Minimize the firefighting. Maximize ROI on pipelines.
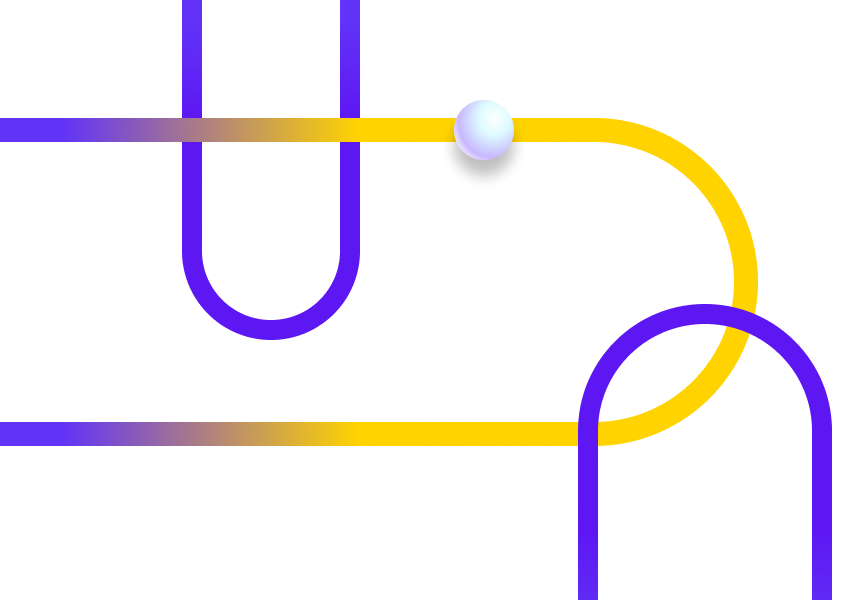
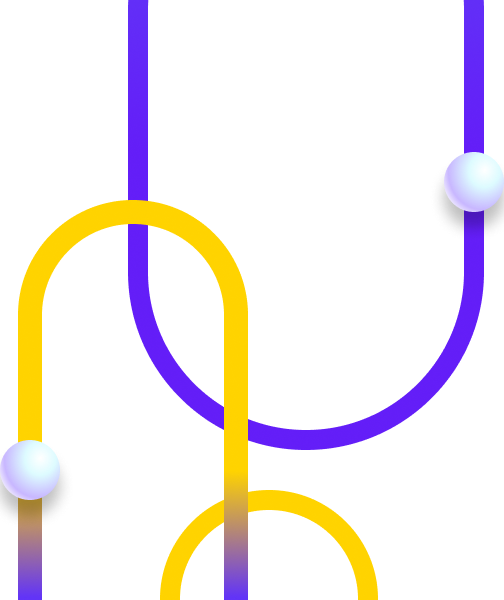