As businesses increasingly rely on data-driven insights, the need for effective data handling has become more pronounced than ever. Therefore, the concepts of data management and data governance have gained prominence, each playing a distinct yet interconnected role.
This article delves into the critical nuances between DevOps and DataOps, shedding light on their key differences and similarities of data management vs data governance. Understanding these distinctions is paramount for organizations seeking to harness the full potential of their data in an era where information is not just an asset but a strategic imperative.
Understanding Data Governance
Data governance serves as the compass guiding organizations in the responsible and effective management of their data assets. At its core, data governance is a set of principles and practices aimed at ensuring the availability, usability, integrity, and security of an organization’s data. It also establishes a framework for decision-making and accountability and defines the rules and policies governing data throughout its lifecycle.
Objectives and Goals of Data Governance
The primary objectives of data governance revolve around optimizing the value derived from data assets while minimizing risks associated with their use. This includes defining and maintaining data quality standards, ensuring data consistency across the organization, and establishing clear ownership for data-related processes.
Ultimately, data governance aims to foster a data-driven culture where decisions are informed, consistent, and aligned with organizational objectives.
How Data Governance Contributes to Regulatory Compliance and Risk Mitigation
By implementing robust data governance practices, organizations can adhere to regulatory requirements, safeguard sensitive information, and reduce the likelihood of data breaches.
Clear policies and procedures, coupled with effective data governance, provide a structured approach to risk management, instilling confidence among stakeholders and regulatory bodies alike.
Navigating Data Management
Data management constitutes the tactical execution of strategies laid out by data governance. It involves the processes and activities aimed at acquiring, organizing, storing, and utilizing data throughout its lifecycle.
The scope of data management encompasses a broad spectrum, ranging from the technical aspects of database administration to the practicalities of ensuring data quality and usability.
Key Components and Activities
Data management involves a series of interconnected components and activities, all aimed at optimizing the use and value of data within an organization. This includes data modeling, database design, data integration, and the implementation of data security measures.
Moreover, data management extends to establishing data quality standards, metadata management, and enforcing data policies. The seamless coordination of these components ensures that data remains accurate, available, and aligned with organizational goals.
Data Management and Organizational Efficiency
At its core, data management is intricately linked to organizational efficiency. Efficient data management ensures that data is readily available to those who need it in an easily understandable and usable format. This enhances operational efficiency and fosters a culture of data-driven decision-making throughout the organization.
As data becomes a more integral part of day-to-day operations, the efficiency gains facilitated by robust data management practices contribute directly to an organization’s overall success and competitiveness in the modern business landscape.
Key Differences: Data Governance vs. Data Management
Let’s see how is data governance different from data management.
Focus and Scope
The primary focus of data governance is on establishing the framework, policies, and standards for managing data assets. It is concerned with ensuring that data is used responsibly, ethically, and in compliance with regulatory requirements. The primary aim is to set the rules and guidelines governing the strategic use of data throughout the organization.
In contrast, data management has a broader scope that encompasses the tactical implementation of the guidelines set by data governance. It involves the day-to-day data-related activities, such as data acquisition, storage, processing, and distribution. While guided by data governance, data management is more hands-on and involves the practical execution of strategies to optimize the use of data.
Objectives and Goals
The specific goals of data governance include defining data ownership, ensuring data quality, and mitigating risks associated with data use. It aims to establish clear accountability for data-related processes, fostering a culture of responsibility and compliance within the organization.
On the other hand, data management focuses on optimizing the efficiency of data processes. Its goals include ensuring data availability, usability, and integrity. Data management aims to streamline data-related activities, making data easily accessible and usable for various business functions.
Stakeholders and Responsibilities
Key stakeholders in data governance include executive leadership, data stewards, and compliance officers. These individuals are responsible for setting policies, ensuring adherence to regulations, and overseeing the ethical use of data throughout the organization.
Data management involves a range of roles and responsibilities. Database administrators, data analysts, and IT professionals play crucial roles in implementing data management practices. Their responsibilities include database maintenance, data integration, and ensuring the day-to-day functionality of data systems.
Understanding the data governance vs data management key differences is essential for organizations aiming to establish a holistic approach to data. While data governance sets the strategic framework, data management executes the tactical processes, collectively ensuring that data serves as a valuable and reliable asset for organizational success.
Aspect | Data Governance | Data Management |
Focus and Scope | Establishes guidelines and policies for strategic data use. | Implements strategies for day-to-day data processes. |
Objectives and Goals | Defines data ownership, ensures data quality, and mitigates risks. | Focuses on optimizing data efficiency, availability, and usability. |
Stakeholders and Responsibilities | Involves executive leadership, data stewards, and compliance officers. | Includes roles like database administrators, data analysts, and IT professionals. |
The Interplay: How Data Governance and Data Management Collaborate
The symbiotic relationship between data management and governance is crucial for organizations seeking to extract maximum value from their data assets. While data governance sets the strategic direction and guidelines, data management puts these into action. Their interconnectedness is vital for creating a seamless and effective data ecosystem within an organization.
The case study of Artlist underscores a triumphant collaboration between data governance and data management driven by Rivery, Snowflake, and Aggua. This transformative journey resulted in a 4X acceleration in time to value, improved data accessibility across the company, and a 70% boost in analysts’ capacity.
With measurable improvements in key metrics and a 5X growth in the data team, Artlist’s story exemplifies how effective data governance and management practices can catalyze a data-driven organizational shift, fostering efficiency, productivity, and strategic decision-making.
It shows that effective integration of data governance and data management involves adopting practices that align the strategic vision with operational execution. This involves proven methods for fostering collaboration, including clear communication channels, cross-functional collaboration, and establishing feedback loops to ensure continuous improvement.
Challenges and Solutions
Implementing robust data governance and data management practices doesn’t come without challenges. Some of the common hurdles organizations face are resistance to change, lack of clear communication, and the complexity of data ecosystems.
Acknowledging these challenges is the first step toward devising effective solutions. Addressing them requires strategic thinking and proactive solutions, from fostering a culture of data responsibility to investing in comprehensive training programs.
Industry Compliance and Regulations
Both data governance and data management play integral roles in ensuring compliance. They collaborate to meet regulatory requirements thanks to a comprehensive approach that aligns strategic governance principles with meticulous data management practices.
Ensuring compliance includes adhering to industry-specific regulations and mitigating risks associated with data use. By integrating robust data governance and management practices, organizations can navigate regulatory landscapes and safeguard against potential risks, ensuring the ethical and responsible use of data.
Future Trends and Innovations
The landscape of data governance and management is continuously evolving. From integrating artificial intelligence and machine learning for data insights to the rise of decentralized data management models, staying abreast of these trends is crucial for organizations aiming to remain at the forefront of effective data handling.
Innovation is the cornerstone of progress in data handling and oversight. Whether it’s the advent of blockchain for enhanced data security or the utilization of advanced analytics for predictive insights, understanding the recent and foreseeing the upcoming innovations is essential for organizations seeking to future-proof their data strategies.
Conclusion
The critical distinctions of data governance vs data management are fundamental for organizations navigating the data-driven landscape. While data governance sets the strategic framework, defining guidelines and policies for responsible data use, data management executes these strategies, focusing on day-to-day processes to optimize data efficiency.
Now, as organizations chart their data journey, it’s not just about understanding these differences; it’s about taking action. Implementing robust data practices requires proactive solutions, from fostering a culture of data responsibility to investing in comprehensive training programs. This is the right moment to seize the opportunity and align your organization with a holistic approach to data. By doing so, you’re not just navigating the present data landscape but actively shaping a future where your data strategies drive success.
Stay adaptive, foster continuous improvement, and embrace innovation. The data landscape evolves, and your organization’s approach to it should, too. Let this be a rallying call to optimize your data handling and governance for sustained success.
Minimize the firefighting. Maximize ROI on pipelines.
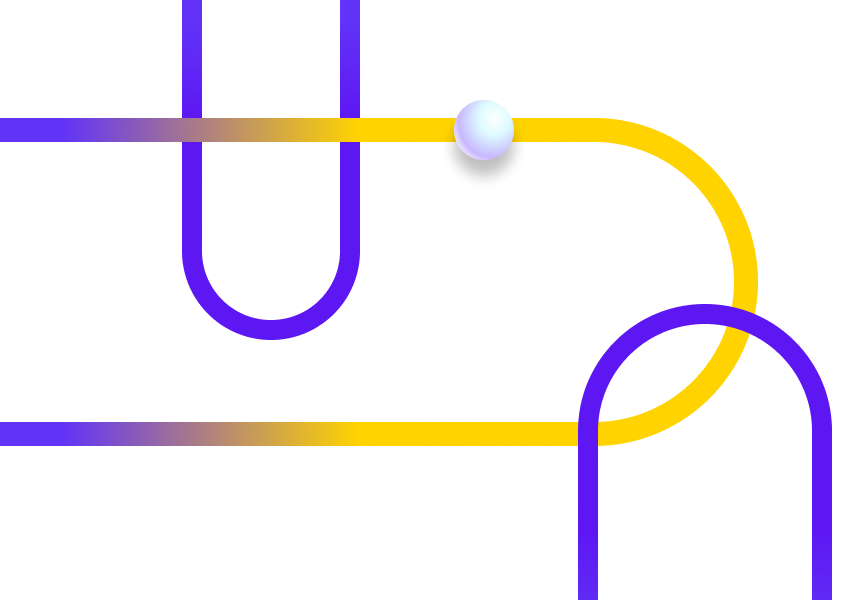
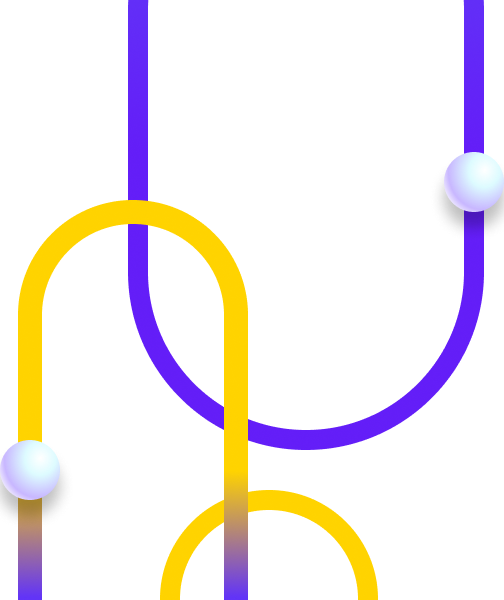