As the amount and variety of data grows exponentially, it becomes increasingly difficult for organizations to manage their data effectively. Organizations that are able to implement an effective data integration approach can empower staff with the right tools and processes to effectively monitor, analyze, and improve performance. This helps organizations make better decisions faster, leading to improved operational efficiency and increased market competitiveness.
Why does it matter, you ask?
Consider this: You are the owner of a bustling pizza chain. Your business has multiple locations, each with its own system for tracking sales, inventory, and customer feedback. Without a sound data integration strategy, you’re left juggling multiple reports, manually comparing numbers, and making educated guesses rather than data-driven decisions.
Now, imagine having a robust data integration strategy in place. All your data, from sales figures to customer preferences, flows seamlessly into a central system. You can instantly see which toppings are most popular, which location is underperforming, or even predict when you’ll need extra staff based on historical sales data. That’s the power of an effective data integration strategy—it turns your data into actionable insights!
Data integration can bridge the gap between siloed business intelligence systems, resulting in better information sharing across departments and improved customer experiences. With a comprehensive data integration strategy, organizations can take advantage of new technologies like machine learning to make well-informed decisions quickly and accurately.
In this blog post, we’ll discuss what is needed to create an effective data integration strategy, the step-by-step process to develop an effective strategy, and provide real-world examples.
Let’s dive right in.
Understanding Data Integration Strategy
A data integration strategy is essentially the roadmap for how you collect, manage, and utilize data across your organization. It sets the stage for how disparate data sources and systems communicate with each other, efficiently combining data from different sources into a unified, easily accessible format.
This strategy is instrumental in ensuring data consistency, accuracy, and reliability, driving informed decision-making, and ultimately propelling business growth. Without a well-considered data integration strategy, organizations risk making decisions based on inaccurate or incomplete data, which can have serious implications for business performance and competitiveness.
Dismantling Data Silos with Data Integration Strategy
One of the major challenges faced by many organizations is the existence of data silos. Data silos refer to situations where only one group or department within an organization can access a set of data or information. This happens most often due to the use of different software systems or platforms that don’t communicate with each other. The consequence? Fragmented insights and a lack of a unified view of the business.
Enter the data integration strategy.
A robust data integration strategy facilitates the seamless sharing and communication of data across disparate systems and departments. Not only does it break down the walls of data silos, but it also creates a unified, cohesive information landscape from which to draw insights.
By efficiently combining and reconciling data from different sources, a data integration strategy provides a 360-degree view of the business. It enables organizations to uncover hidden patterns, detect trends, and make accurate predictions. This deep level of insight is impossible to achieve when data is scattered and disconnected.
Think of your data integration strategy as the maestro of an orchestra. Each instrument (or data source in our case) plays its part, but the magic truly happens when they all come together in harmony, creating a symphony of actionable business insights.
- Enhances data accuracy and consistency: A robust data integration strategy ensures all departments use the same data, promoting cohesive decision-making across the organization.
- Increases operational efficiency: Automating data collection and aggregation allows your team to focus on value-adding tasks like data analysis and strategy development.
- Enables faster, informed decisions: With a comprehensive view of all your data, you can quickly identify trends, forecast outcomes, and gain a competitive edge.
- Improves customer experience: Integrating data from various touchpoints provides a holistic view of your customer’s journey, helping to tailor offerings, enhance satisfaction, and foster loyalty.
- Lays foundation for advanced technologies: A strategic approach to data integration prepares your business for utilizing AI and machine learning, which can generate accurate predictions, provide deeper insights, and drive growth.
In essence, the benefits of a well-crafted data integration strategy go beyond just data management. It is a key driver of operational efficiency, informed decision-making, customer satisfaction, and innovation. It’s the secret sauce to turning your data into a powerful business asset.
Key Requirements for Data Integration Strategy
To ensure your data integration strategy delivers the expected results, you need to get a few key elements right. These are:
Data Quality: This is the bedrock of any successful data integration strategy. Data should be clean, accurate, and consistent to generate reliable insights. Implementing quality checks at each stage of the integration process can help ensure high-quality data.
Compatibility and Scalability: Your data integration solution needs to be compatible with the various types of data sources in your ecosystem. Additionally, it should be scalable to accommodate growth in data volume and complexity over time.
Security and Compliance: Protecting sensitive data during the integration process is critical. The solution should include robust security measures and comply with relevant data privacy regulations. Regular audits and updates can further enhance data security.
Real-World Consequences of Inadequate Requirements in Data Integration Strategies
Let’s consider some real-world examples that illustrate the consequences of not adequately addressing these key requirements in your data integration strategy.
Example 1: Poor Data Quality
Gartner recently discovered many businesses lose thousands of dollars each year as a result of “lost productivity” stemming from poor-quality data. Poor data quality is considered responsible for costing organizations an average of $15 million per year.
A leading eCommerce brand noticed a significant decline in its campaign performance. After an internal audit, the company discovered discrepancies in its customer database – duplicate entries and outdated customer contact details. The poor data quality led to ineffective marketing strategies and wasted resources, leading to the decline in their campaign performance. This could have been avoided with a robust data integration strategy that emphasizes data quality.
Example 2: Lack of Compatibility
A healthcare organization adopted a new Electronic Health Record (EHR) system, but it was incompatible with their existing databases. This incompatibility hindered the smooth flow of patient information across departments, resulting in inefficient operations and poor patient care. The organization had to invest additional resources to resolve this issue.
A better approach would have been to consider the compatibility aspect in their data integration strategy from the beginning. Today, interoperability standards such as FHIR and HL7 are a must for any healthcare organization looking to integrate multiple systems.
Example 3: Ignoring Security and Compliance
A significant security incident has impacted Deutsche Bank, ING Bank, Postbank, and Comdirect. These banks reported customer data leaks from the same third-party vendor, Majorel, which was breached in the Cl0p attacks. The breach was a result of a hack on MOVEit, a (supposedly secure) file transfer technology.
These examples highlight the potential pitfalls of overlooking the key requirements in your data integration strategy, further underlining the importance of getting these elements right.
The Data Integration Process: Step-by-Step
With the requirements in mind, let’s walk through the steps involved in crafting an effective data integration strategy.
Navigating the journey of data integration is akin to weaving together different threads to create a coherent tapestry.
Successful data integration means that different data sources can effectively “speak” to each other, paving the way for holistic insights and smarter decision-making. Let’s delve deeper into each of these steps, unpacking their significance and the challenges often faced:
1. Data Extraction: Gathering Insights from Various Sources
Data extraction from multiple sources, such as databases, flat files, APIs, and other platforms, is the first step in bringing disparate data together.
Challenges: Ensuring that data from diverse sources is accessible, accounting for different data formats, and managing potential changes to the source systems.
2. Data Transformation: Shaping Raw Data into Valuable Insights
Raw data often comes in formats and structures that might not be suitable for direct analysis. Data transformation helps refine and reshape this data for the target system or analysis.
Challenges: Handling inconsistencies, dealing with missing values, and defining business rules for transformation. This step also includes potential normalization, enrichment, and data cleansing activities.
3. Data Delivery: Establishing Trust Between Systems
Once data is extracted and transformed, it needs to be delivered to its target destination. This is not just a technical challenge; it also means ensuring that data remains secure and compliant during transfer.
Challenges: Security concerns, dealing with potential data breaches, ensuring data encryption, and meeting regulatory compliance standards.
4. Data Loading: The Bridge to Seamless Data Flow
Loading data refers to the process of moving the transformed data to its final destination, often a database or data warehouse. This can be done in batches or real-time, depending on the needs of the organization.
Challenges: Optimizing for speed to avoid bottlenecks, handling large volumes of data, and managing failures or errors during the loading process.
5. Data Validation: Ensuring Accuracy and Reliabiliy
Validation ensures that the data being integrated adheres to predefined standards and rules, ensuring accuracy and reliability. This step is crucial in maintaining data quality.
Challenges: Setting up automated checks and balances, handling exceptions, and managing data quality over time as more data gets integrated.
6. Process optimization: Iterative improvements for sustained success
After the initial data integration process is over, it’s important to ensure that its performance and results are monitored closely. Optimization helps maintain the accuracy of the data while increasing efficiency.
Challenges: Identifying potential areas for improvement, using automation and AI for optimization, and staying up to date with emerging technologies.
Real-life Examples of Successful Data Integration
Data integration can provide significant benefits across various sectors, from healthcare to consumer packaged goods. Here are some real-world examples demonstrating the transformative potential of effective data management.
Healthcare: Better Research Outcomes
In the healthcare industry, organizations often face challenges in managing vast amounts of data from various sources. Bayer, a leading pharmaceutical company, effectively improved its data granularity and control by implementing a comprehensive data integration strategy. They needed instant access to all current data sources and the ability to easily add new ones. By leveraging a platform with over 200 native connectors, Bayer could instantly connect to required sources and then add many more, including Twitter and Snapchat.
This data integration strategy enhanced transparency and provided more granular data, enabling Bayer to handle an increased volume of data. Within six months, Bayer boosted its data usage on the platform by 30% and improved efficiency through automation capabilities. The adoption of this data strategy now allows Bayer to manage all its marketing data and connect with various sources seamlessly.
Manufacturing: Increased Efficiency and Productivity
The manufacturing sector often relies heavily on manual processes, resulting in errors and delays. A global food manufacturer and Fortune 500 company was faced with the challenge of launching a new health-based food brand. The team, though part of a well-established conglomerate, had the mindset of a startup – limited resources, a compressed timeline, and no brand recognition. They needed a data infrastructure that could handle a vast amount of logistical and marketing data associated with launching a new product directly to consumers.
The company sought an out-of-the-box solution that would allow them to connect readily to all their data sources without requiring extensive coding or developer resources. They wanted to maintain full control over their data and “own the pipes” of their data infrastructure to scale rapidly.
The solution they chose enabled them to connect to their data sources within minutes, providing immediate value. It also came with a customer success team that acted as a data engineer at their disposal.
The result was a flexible, cost-effective solution that allowed the team to hit the ground running, tracking everything from ad spend to packaging. They were able to scale from day one while maintaining the standards required of a Fortune 500 company, including data security and legal compliance.
SaaS: Enhanced Customer Experience
Artlist, a creative technology company serving clients like Google, Apple, and Nike, faced the challenge of quickly consuming data from multiple sources for their fast-growing subscription-based product. Their small data team struggled to build end-to-end data pipelines, affecting their ability to deliver business insights rapidly.
Rivery, Snowflake, and Aggua provided the solution to Artlist’s data challenges.
Rivery’s simple setup, native connectors, and ready-made data model templates eased data collection from various sources like Facebook and Google Ads. This enabled daily consumption of product usage and marketing data by different teams. Aggua, a Rivery partner, helped visualize data lineage, making it easier for analysts to understand the data they were working with.
As a result, Artlist saw a 60% boost in time to value, a five-fold increase in data team size, and a 70% increase in the analysts’ capacity to answer key business questions. R&D time was freed by 50%, and resources were saved due to minimal onboarding for new data team members. Rivery’s no-code solution transformed Artlist into a more data-driven organization.
Best Practices for Implementing Data Integration Strategy
Plan a Coherent Strategy: A well-planned strategy considers all aspects of data integration, including data governance, cloud service providers, and the selection of a data integration provider.
Analyze Data Sources: Begin by listing all data sources you plan to use. This includes internal databases, external APIs, SaaS applications, and other third-party sources. Ensure these sources are compatible with each other and can scale with your growth.
Set Clear Goals and Metrics: Identify the specific purpose of the data integration. Are you looking to improve customer experience, automate operations, or gain a competitive edge? Setting clear goals will help you stay focused on what’s important and choose the right approach for your needs.
Design Data Model: This involves creating a detailed data model to define the structure and flow of data between different sources. You need to consider factors like data organization, data latency, and accessibility.
Choose the Right Approach and Platform: Different data integration approaches suit different business needs. It’s important to choose an approach that aligns with your business goals. The platform should be scalable, secure, and easy to use.
Simplicity is Key: Minimizing complexities in data integration techniques can help speed up the process and reduce errors.
Ensure Data Quality and Security: Data quality management and enhanced security measures are crucial in big data integration.
Conduct Thorough Testing: Testing the data integration process helps identify and rectify issues before they impact business operations.
Embrace Flexibility and Adaptability: Dynamic data landscapes require flexibility and adaptability. Be prepared to adjust your strategy as business needs, technologies, and data sources evolve.
Monitor and Optimize: Constant monitoring will help identify potential issues, allowing you to optimize your data integration strategy for better performance.
Remember, a successful data integration strategy requires careful planning, execution, and ongoing management. It’s not just about the technology but also how it’s used to meet business objectives.
The Bottom Line
Navigating through the intricacies of data integration strategy can seem daunting, but as our journey through real-world examples and best practices has shown, it’s a rewarding expedition.
The importance of a well-structured data integration strategy cannot be overstated. It serves as the backbone of your organization’s data infrastructure, determining the efficiency, security, and overall success of your data operations.
Equipped with the knowledge from this guide, you are empowered to plot your own course in the data integration landscape. Remember, it’s not just about the technology but also how it’s leveraged to meet your unique business objectives.
Further Reading and Resources
Books on Data Integration: For a comprehensive understanding of data integration, consider reading “Data Integration Blueprint and Modeling” by Anthony David Giordano, and “Enterprise Data Integration: A Practitioner’s Guide to Techniques and Tools” by Rob P. Ruel, A.J. Stuyt, and Erik Fransen.
Online Courses: Websites like Coursera, Udemy, and edX offer numerous online courses that delve into data integration strategies. These utilize real-world examples and case studies, providing practical knowledge on the subject.
Blogs and Articles: For ongoing learning and up-to-date information, follow industry-leading blogs like Rivery, Datafloq, Dataversity, and Towards Data Science. These sites regularly publish articles on data integration strategies and other related topics.
Webinars and Conferences: Participating in webinars and conferences can provide valuable insights from industry experts. Keep an eye on events hosted by organizations like Gartner, Forrester, and IDC.
Remember, the world of data integration is vast and evolving. Constant learning and adapting to new trends, tools, and techniques is key to successfully implementing your data integration strategy.
Minimize the firefighting. Maximize ROI on pipelines.
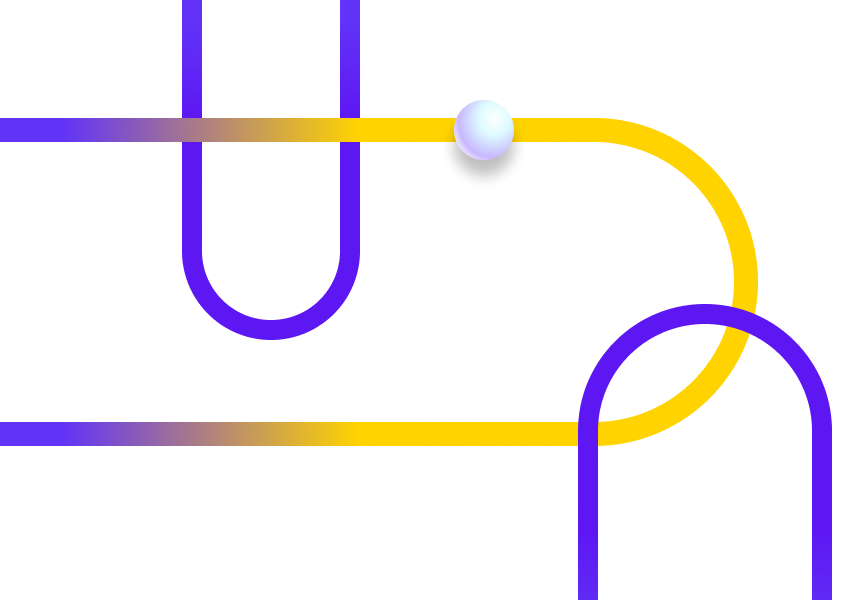
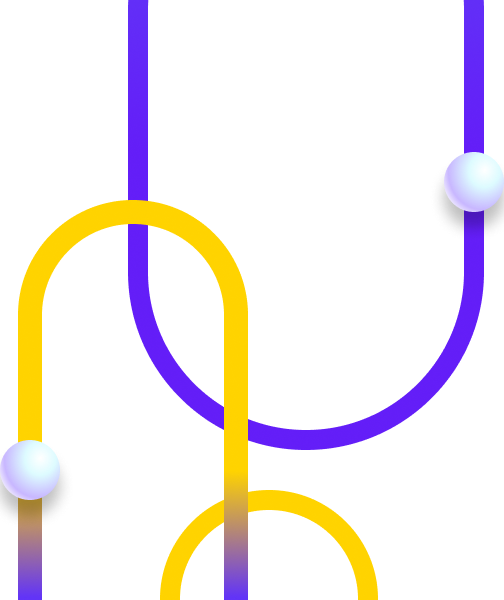