In today’s data-driven world, businesses are overloaded with vast information and trying to make sense of it all. Data comes in various forms, and understanding its nature is crucial for effective data management and maintaining productivity.
There are two main categories for data storage and information processing – structured and unstructured data. What is the main difference between structured and unstructured data? Recognizing their differences is essential for your data operations, making informed decisions, and getting valuable insights.
In his article, we’ll dig into some prime examples of structured and unstructured data, to help you understand their core characteristics, use cases, and benefits.
Definition of Structured Data
Structured data is information presented in a well-defined format, making it easily identifiable and searchable. This type of data is usually organized into tabular forms (rows and columns) and provides better information storage and analysis, often referred to as schema-on-write.
Definition of Unstructured Data
Unstructured data is information that is not presented in a predefined structure or format and is not processed until it is ready to be used. It is commonly referred to as schema-on-read. This information is not written into ‘’traditional’’ rows and columns that are a common part of a predefined schema.
Importance of Understanding the Differences Between Structured and Unstructured Data
Knowing the difference between structured and unstructured data can affect how businesses manipulate, store, process, and analyze information. This know-how can help organizations properly plan and prepare their strategies, assess and decide on the technologies and tools they need to use to manage data more efficiently. We’ll dive into structured vs unstructured data examples further on in the article to help you understand their differences better.
Characteristics of Structured Data
Structured data has characteristics that set it apart from other data types. For example, it is easily used by machine learning algorithms, has an easily identifiable structure, is stored in data warehouses, and makes use of SQL relational databases.
Well-Defined and Organized Format
Structured data is quantitative data presented in a well-defined and organized format, making it easily readable by machine learning algorithms. It is formatted into standardized data models that enable efficient querying and analysis and enforce data integrity rules.
Examples of Structured Data Sources
Structured data is often generated into a specific schema or data model, like spreadsheets or XML files. Examples of structured data include transactional databases, customer relationship management (CRM) systems, financial records, invoices, and log files.
Common Data Models Used for Structured Data
Hierarchical, relational, and network databases are common types of data models used for structured data.
Characteristics of Unstructured Data
Unstructured data, generated in its native or raw form, is more challenging to analyze and organize compared to structured data. It makes use of NoSQL non-relational databases, is usually stored in data lakes, and has faster accumulation rates.
Lack of Predefined Structure or Format
With the unstructured data, there is a lack of predefined structure or format. This disadvantage makes the job more challenging for businesses to organize and analyze their information.
Examples of Unstructured Data Sources
Unstructured data is qualitative data in the form of free text, multimedia content, posts on social media, emails, documents, images, videos, customer reviews, and other data types that do not have a standardized organization.
Challenges in Processing and Analyzing Unstructured Data
The volume of unstructured data, the variety of information, and the lack of predefined structure make it difficult to turn the data into meaningful insights and challenging for advanced technologies like ML and natural language processing.
Key Differences between Structured and Unstructured Data
Structured and unstructured data are different in many ways. Here are some of the key differences.
Data Organization and Format
Structured data is presented in predefined tables, while unstructured data is not. Therefore, structured data is more suitable for traditional data management, where efficient storage and analysis can be made. On the other hand, unstructured data lacks specific information organization and requires the use of more specialized techniques to extract valuable information.
Data Storage Requirements
When it comes to information storage, usually structured data is stored in data warehouses. These are storage systems with a predefined format. However, if changes are made, all of the structured data will be updated according to the requirements, which will cause the expenditure of resources and time. To mitigate costs and achieve better scalability, companies use cloud-based data warehouses.
Unstructured data and its lack of structure require the use of flexible storage systems, like cloud data lakes, object storage solutions, or file systems.
Data Analysis and Processing Techniques
Structured data can be easily analyzed using data mining methods and traditional statistical techniques like SQL queries. Unstructured data needs advanced technologies such as NLP, text mining, and ML.
Scalability and Flexibility
Structured data has limited scalability because of its predefined schemas and fixed structure. Unstructured data is highly scalable and can adjust to any data without changing the existing structure.
Use Cases and Applications
Structured and unstructured databases have different use cases and applications across various industries.
Industries and Scenarios Where Structured Data Is Prevalent
Structured data is used in retail, telecommunications, finance, healthcare, and manufacturing industries. Due to its set structure, it’s often employed for CRM, online booking, or accounting analysis.
Industries and Scenarios Where Unstructured Data Plays a Significant Role
Unstructured data is the best choice for industries such as data mining, chatbots, social media, and marketing. It can help make predictive data analysis and adjust according to market shifts.
Real-World Examples Highlighting the Differences in Data Types
For example, structured data can be used for financial databases where transaction records are well organized into a tabular form where the specific attributes are represented and ready for processing and analysis.
On the other hand, if we look into an example in the marketing field where unstructured data is used, we can see that it is a collection without a predefined format of social media posts where customers have shared their experiences.
Structured vs Unstructured Data Integration
The integration of structured and unstructured data, known as semi-structured data, is the bridge between both of them. The idea of merging structured and unstructured data is to improve analysis and efficient decision-making.
Strategies for Integrating Structured and Unstructured Data
Businesses can implement structured and unstructured data by employing data integration like data warehousing, data lakes, and data virtualization. Combining structured and unstructured data sources can increase the productivity and efficiency of the analysis.
Tools and Technologies for Handling Both Data Types
There are a number of tools and technologies that support the analysis and management of structured and unstructured data. Structured data is usually handled by RDBMS like MySQL, PostgreSQL, and Oracle, while unstructured data requires technologies such as NoSQL databases, Apache Hadoop, Apache Spark, and Elasticsearch.
Challenges and Considerations in Combining Structured and Unstructured Data
Combining structured and unstructured data is quite a challenge to maintain data quality, data performance, and scalability. In this case, businesses should establish data governance practices, use the proper tools and technologies, pay attention to the specific requirements of both data types, and ensure successful data integration.
Impact of Structured and Unstructured Data on Decision-Making
Structured and unstructured data have a significant impact on the decision-making process.
How Structured Data Supports Traditional Analytics and Reporting
Structured data plays a huge role in managing traditional analytics and reporting within business activities. It supports the consistent data structure, and improves the efficiency of data storage and retrieval. It can be used in standardized querying and analysis, enhance data consistency and accuracy, provide automotive reporting, and is valuable for capturing historical records.
By manipulating structured data, businesses extract important insights, track the indicators and metrics, monitor the organization’s performance, and deliver data-driven solutions.
How Unstructured Data Enables Insights and Innovation
Even though the unstructured data is not presented in a predefined format, it’s still important and valuable for gathering insights and encouraging innovation within the companies. It plays an essential role in collecting rich sources of information, providing text analytics and NLP, social media listening and sentiment analysis, data mining and pattern recognition, forecasting, and preparing predictive analytics.
By analyzing unstructured information, businesses can discover hidden patterns and trends that may not appear in structured data formats.
If unstructured data is properly analyzed and interpreted, it can deliver a whole set of productive ideas and innovative solutions and have a comprehensive understanding of user preferences, market dynamics, and business opportunities.
The Value of Combining Structured and Unstructured Data for Holistic Decision-Making
Integrating structured and unstructured data offers a whole new holistic perspective on the organization’s landscape. By combining both, businesses can uncover critical insights and valuable correlations that will lead to making informed decisions. Due to the synergy between them, businesses can gain more knowledge based on various information sources.
Data Management and Governance Considerations
Proper data management and governance are vital for businesses to ensure data collection’s integrity, quality, and security.
Data Management Practices for Structured Data
Structured data requires effective data management practices, such as data modeling, validation, cleansing, and metadata repositories. Common practices are data governance, modeling, data quality management, data backup and recovery, data retention and archiving, and data lifecycle management.
Data Management Challenges for Unstructured Data
Most of the data management challenges for unstructured data are caused by its lack of structure. Key challenges associated with unstructured data management are data volume and variety, data extraction and transformation, metadata management, data quality and consistency, data integration, data storage and scalability, data governance, and data retention.
By overcoming these challenges, businesses can unlock various options on how to process the information and deliver better solutions.
Approaches to Data Governance for Both Data Types
Propper data governance in both structured and unstructured data can be established by implementing data governance frameworks, policies, and protocols to ensure information quality, security, and compliance. To accomplish this, businesses should implement data access controls and data lifecycle management practices.
Future Trends and Opportunities
The science behind data management is constantly evolving, and new opportunities and trends should emerge for better success and business productivity.
Advancements in Structured Data Management and Analysis
Businesses will continue to work and invest in data integration technologies to streamline the process of integrating structured data from different sources. These technologies will give a better structure and unified view of structured data, reducing data silos and providing real-time access and analysis.
Organizations will continue to invest in data integration and virtualization technologies to streamline the process of combining structured data from disparate sources. These technologies will provide a unified view of structured data, eliminating data silos and enabling real-time access and analysis. Also, cloud-based data management, DataOps, and agile data management will continue to dominate the data management landscape.
Emerging Technologies for Unstructured Data Processing
Emerging technologies like deep learning, NLP, and computer vision will still be imperative for efficient data management. Speech recognition and voice analytics are new segments that will also be used in unstructured data processing, enabling businesses to get insights from customer service and call centers and use voice-based virtual assistants.
The Convergence of Structured and Unstructured Data
Integrating structured and unstructured data is getting more and more popular. Having the best of both worlds will boost businesses’ confidence regarding data manipulation, decision-making, and implementing creative solutions.
The advantages of merging structured and unstructured data are better data fusion and integration, advanced analytics and AI, and comprehensive contextual analysis and personalization. These technologies will improve the accuracy and effectiveness of tasks like photo recognition, understanding of natural language, and defect detection.
Conclusion
Structured and unstructured data are two terms that are significantly different in terms of their organization, storage requirements, processing and analysis techniques, and applications.
Understanding the differences is essential for businesses to properly manipulate the database, conduct impactful analysis and make the right decisions. By combining the strengths of structured and unstructured data, businesses can achieve a comprehensive perspective of their operations, users, and market dynamics.
Minimize the firefighting. Maximize ROI on pipelines.
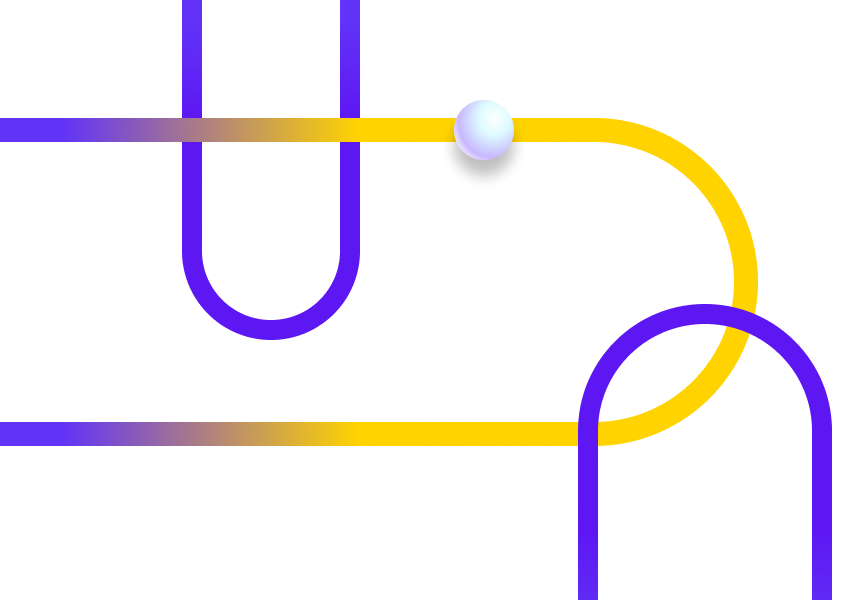
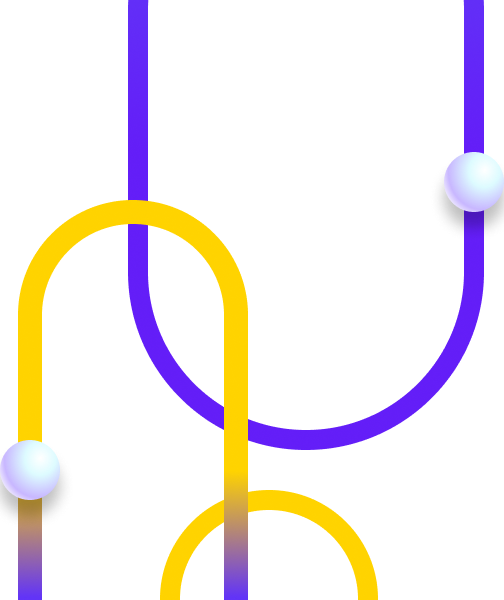