In the rapidly evolving digital age, data has transcended its role as mere information to become the lifeblood that propels organizations forward. As businesses navigate the complexities of the modern era, the skillful management, analysis, and extraction of insights from colossal datasets have become advantageous and paramount for success.
The DataOps architecture emerges as a transformative force in response to this data-centric paradigm. This innovative approach represents a shift in how businesses conceptualize and handle their data.
What exactly is DataOps, and why has it risen to such prominence? Join us as we demystify the intricacies of DataOps, exploring its significance and principles, the data architecture components, as well as the tools to not just adapt to the data-driven landscape but thrive in it.
Understanding DataOps
DataOps is an agile, cross-functional methodology designed to improve the speed, quality, and reliability of data analytics. It combines aspects of Agile development, DevOps, and statistical process controls to provide high-quality, reliable data at a rapid pace.
At its core, DataOps focuses on automating data flows, orchestrating data across multiple platforms, and fostering collaboration between teams that handle data. It seeks to break down silos that often exist between operations, development, and business teams, enabling them to work together more effectively.
The significance of DataOps lies in its potential to address many of the challenges businesses face with data management. From eliminating bottlenecks and reducing errors to ensuring compliance and improving data quality, DataOps offers a holistic solution to many common issues.
Successful Outcomes from Adopting DataOps Architecture
Embracing DataOps yields transformative outcomes across diverse sectors. In content streaming, organizations leverage DataOps to refine content delivery through real-time integration of user preferences, enhancing their competitive edge.
Music streaming platforms orchestrate vast music data using DataOps, tailoring recommendations and playlists for individual users. The financial sector accelerates data insights by automating processes, facilitating quicker and more informed decision-making.
Social media entities employ DataOps for managing massive user data, optimizing algorithms to deliver relevant content, and boosting engagement. In e-commerce, DataOps refines operations by integrating data from various sources, enhancing responsiveness and efficiency.
This underscores the universal impact of DataOps in achieving efficiency, precision, and innovation across industries.
Initiating DataOps
Embarking on a DataOps journey involves a systematic process for optimal results. Here are the key steps to initiate DataOps effectively:
- Thorough Assessment: Conduct a comprehensive evaluation of current data processes. Identify bottlenecks, inefficiencies, and areas for improvement.
- Define Roles and Responsibilities: Clearly define the roles and responsibilities of all stakeholders involved in data operations. This includes technical staff like data engineers and scientists, as well as business users relying on data for decision-making.
- Cultural Transformation: Build a supportive culture and promote values such as transparency, collaboration, and continuous learning. Foster an environment where mistakes are seen as opportunities for growth rather than failures.
Implementing DataOps Practices
Incorporate DataOps practices into your existing workflows by automating repetitive tasks, implementing continuous integration and delivery (CI/CD) pipelines, and using version control for data models.
You might find you can automate certain tasks or redesign processes to be more efficient. Optimizing your data processes through a DataOps lens can help you identify and eliminate bottlenecks, further enhancing efficiency
Case Studies
When exploring data architecture components, real-world examples underscore the transformative impact of DataOps on overall business operations. Take the case of Bayer, a pharmaceutical giant that sought to enhance its data accessibility. Bayer achieved a remarkable feat with Rivery by connecting to 30% more sources within just six months.
The implementation replaced opaque ETL processes with transparency, addressing data connectors and quality challenges. This not only streamlined data orchestration but also highlighted the pivotal role of DataOps architecture in overcoming challenges faced by large-scale enterprises.
Similarly, Alison AI, an AI-powered video creative analysis platform, faced the challenge of managing third-party data for AI-driven insights. By embracing Rivery’s DataOps engine, Alison AI significantly reduced the time spent on tasks, automating critical processes like client onboarding and historical data retrieval.
The implementation showcased the scalability of DataOps, enabling Alison AI to apply solutions across hundreds of clients seamlessly. From reducing hours to minutes in automating critical processes to saving costs on FTE, the positive outcomes exemplify the tangible benefits of DataOps in a dynamic business landscape.
Key Takeaways
These real-world examples highlight the intersection of DataOps and DevOps, emphasizing the importance of streamlined collaboration between data and development teams.
In both cases, the implementation of DataOps processes led to a cultural shift, fostering a more collaborative and efficient working environment. This accelerated data-driven decision-making and showcased the crucial synergy between DevOps and DataOps methodologies.
In essence, these examples underscore that efficient data management, facilitated by DataOps, is a cornerstone for businesses seeking agility, innovation, and improved operational outcomes in the digital age.
Overcoming Challenges
Despite its many benefits, adopting DataOps can present certain challenges. However, with effective change management strategies, adequate training, and the right tools, these challenges can be overcome.
Resistance to Change
Employees accustomed to traditional data management processes may resist the shift to DataOps. Effective change management strategies, including training programs and clear communication, can foster a culture where the benefits of DataOps are understood and embraced.
Skills Acquisition
Implementing DataOps may require a specific skill set that organizations might lack initially. Investing in training programs or hiring individuals with expertise in both data management and agile methodologies can mitigate this challenge.
Technical Complexities
Integrating various tools, managing data pipelines, and ensuring seamless team collaboration can be challenging. Utilizing comprehensive planning, well-defined processes, and tools that offer flexibility and scalability can simplify them.
Future Trends in DataOps
The DataOps field is continually evolving, with several trends on the horizon. These include the increasing use of artificial intelligence and machine learning in data operations, the rise of real-time data analytics, and the growing importance of data governance.
Staying abreast of these trends can help organizations prepare for the future of data management. By embracing innovations and continuously improving their data operations, they can stay ahead of the curve and maintain a competitive edge.
Conclusion
In conclusion, DataOps architecture offers a powerful approach to managing and leveraging data in today’s fast-paced, data-driven world. It promises improved data quality, increased efficiency, and enhanced collaboration, among other benefits.
Organizations can significantly enhance their data efficiency and derive greater value from their data by understanding what DataOps is, recognizing its benefits, and learning how to implement it effectively. So why wait? Start exploring the potential of DataOps today!
Minimize the firefighting. Maximize ROI on pipelines.
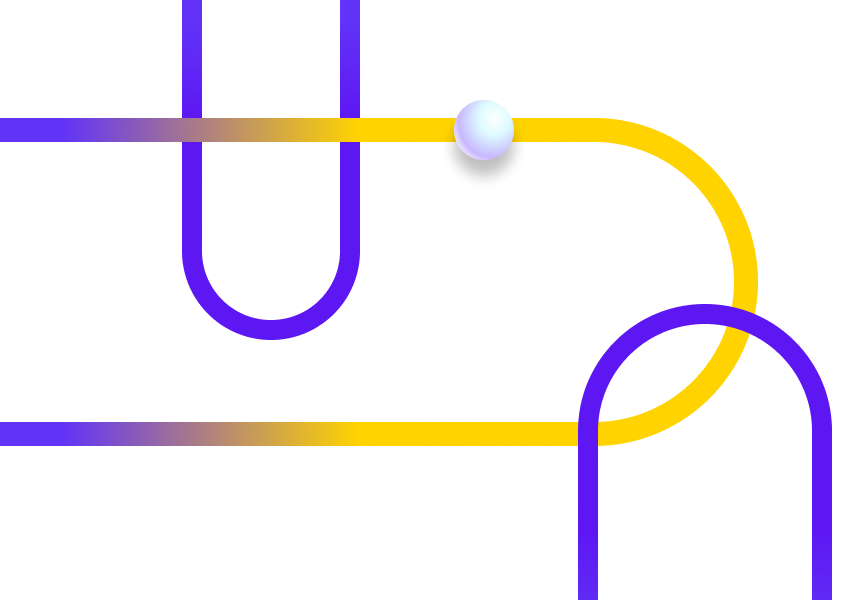
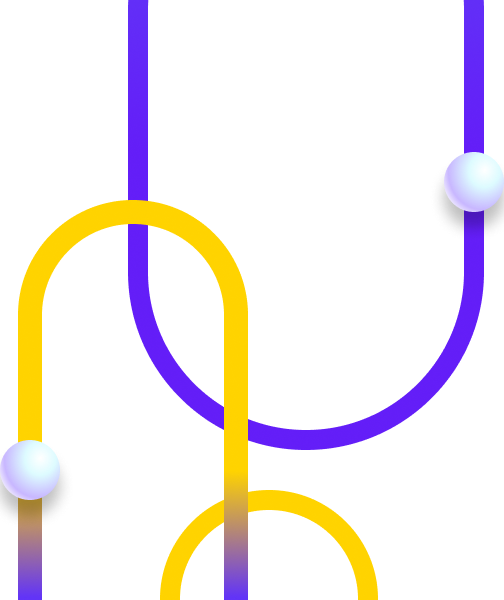