DataOps is all the rage in the tech and business world. But why is it so important? Well, let’s start with a quick refresher on what DataOps actually entails.
In simple terms, DataOps is an agile approach to data management that covers every aspect – people, processes, and technology – to deliver valuable insights from data quickly and reliably. DataOps is an automated, process-oriented methodology that improves the quality and reduces the cycle time of data analytics. The DataOps approach combines Agile development, DevOps, and automated workflow to provide high-quality, reliable data analytics at speed.
Understanding the Traditional Data Pipeline
Traditionally, data pipelines involve a series of stages, from data collection and ingestion to processing, storage, analysis, and visualization. Each stage is rife with potential challenges:
- Data might come from disparate sources and in different formats, and integrating all these disparate sources into a continuous data pipeline can be challenging
- Transformation processes require a lot of computational power and might be hindered by inefficiencies or bottlenecks.
- A lack of standardized practices across different teams leads to silos, miscommunications, and delays
These challenges often extend the timeframes required for data to move from its raw state to a form where it can generate meaningful insights.
Benefits of DataOps
Now that we have a basic understanding of DataOps let’s dive into its significant benefits for modern businesses. Here are some of the most notable advantages of implementing DataOps practices:
Improved Data Quality: With DataOps, the focus is on continuously improving data quality throughout the entire data lifecycle.
Faster Time to Insight: DataOps accelerates data pipelines, turning raw data into actionable insights at unprecedented speeds.
Enhanced Collaboration Between Teams: One of the core objectives of DataOps is to break down silos and foster collaboration between different teams involved in data management.
Cost Reduction: By automating processes and improving efficiency, DataOps helps organizations save costs on data management.
Scalability & Flexibility: With its agile principles, DataOps can easily adapt to changing business needs, making it a valuable asset for growing businesses.
Improved Data Quality
Accurate data is the foundation of any data-driven decision-making process. Without accurate data:
- When decisions are based on flawed or misleading information, it can easily lead to negative business outcomes.
Predictive models or analytics might produce skewed results, rendering the insights produced unreliable. - Data-driven operations and processes can break down completely, leading to disruptions in business activities.
Ensuring data accuracy is vital for the success of any organization, and DataOps comes into play by providing a robust framework for maintaining accurate and high-quality data. With DataOps, the focus is on continuously improving data quality throughout the entire data lifecycle. By implementing automated testing and monitoring processes, organizations can ensure that their data is accurate, complete, and consistent.
DataOps adopts a proactive approach to data quality. Instead of just fixing problems as they occur, it seeks to prevent them from happening in the first place. Here is how it works:
- Continuous Monitoring: Instead of occasional checks, DataOps emphasizes real-time or near-real-time continuous monitoring of data sources, pipelines, and processes. This ensures that any anomalies, inconsistencies, or errors in the data are detected as they arise, empowering organizations to take immediate corrective action.
- Automated Validation: DataOps tools often incorporate automated validation checks and automated testing to validate data at every stage of its journey, from initial collection and processing to analysis and reporting. These checks can identify missing data, outliers, or values that don’t conform to predefined criteria or business rules, ensuring that the data is consistent and reliable. This not only maintains the health and reliability of the data but also significantly reduces the time and resources spent on troubleshooting.
- Feedback Loops: DataOps promotes continuous feedback loops, where issues detected during monitoring and validation are communicated back to the teams responsible. This immediate feedback ensures that errors are not just identified but also rectified and the root causes are analyzed to prevent their recurrence.
Faster Time-to-Insight
Speed is of the essence in today’s fast-paced business environment. The real value of data doesn’t lie in the data itself, but in the insights the business can derive from it. The quicker a business can interpret and act on its data, the better equipped it is to adapt to market changes.
This is where DataOps comes into play. One of the standout advantages of DataOps is its ability to significantly speed up data pipelines, ensuring that raw data is transformed into actionable insights in the shortest time possible.
The Outcome: Swift Transformation of Raw Data into Insights
With DataOps, raw data can be ingested, processed, and analyzed quickly and efficiently, ensuring that businesses have access to timely insights. In a world where business dynamics can change rapidly, this acceleration can provide companies with a competitive edge, allowing them to respond to market shifts proactively. By leveraging DataOps, organizations can speed up their decision-making processes, making them more agile and responsive.
Take, for example, Cignal.io, an AI and machine-learning ad tech company. As they scaled up their operations, the real-time querying of over 200 data points from various advertising entities became more challenging. With Rivery.io, they’ve built a serverless data pipeline that unifies Cignal.io’s internal data from different locations. By implementing DataOps best practices, they created a centralized platform for data analysis, gaining access to meaningful insights that were previously beyond reach.
Enhanced Collaboration
In many organizations, the journey of data from collection to actionable insights is a multi-step process involving various teams, each with its specialized skills and functions. This segmented approach has often resulted in silos where each team operates in isolation, leading to inefficiencies, redundancies, and, at times, conflicting methodologies.
One of the core objectives of DataOps is to break down silos and foster collaboration between different teams involved in data management. By bringing together data engineers, data scientists, and business analysts, DataOps promotes a more cohesive approach to data analytics, leading to better overall results.
How DataOps Fosters Collaboration among Cross-functional Teams
Unified Vision: DataOps creates a shared understanding of the organization’s data goals, ensuring that all teams, whether data engineers, data scientists, or business analysts, are aligned in their objectives.
Integrated Workflows: Instead of each team having its own distinct processes, DataOps promotes integrated workflows that encourage inter-team interactions, shared responsibilities, and joint problem-solving.
Shared Platforms and Tools: DataOps often leverages platforms that are accessible to all relevant teams, allowing everyone to view, process, and analyze data in a unified environment.
Continuous Feedback: Built-in feedback loops ensure that insights, challenges, and solutions are shared across teams, promoting continuous improvement and mutual learning.
For example, Webedia, a global media and technology company, needed a centralized data solution to provide insights across its organization. Building a central solution was vital as data influences Webedia’s business decisions, marketing, and monetization strategies. Rivery’s platform ensured all teams, from executives to marketing, had access to essential data. The implementation of a centralized data solution with Rivery resulted in enhanced collaboration between Wrbedia’s data team, internal IT teams, and partner companies’ data engineers.
Cost Reduction
The costs associated with inefficient data management can be staggering. DataOps, with its emphasis on automation, collaboration, and continuous improvement, emerges as a game-changer in optimizing data operations and significantly reducing costs.
How DataOps Optimizes Data Operations and Cuts Costs
Automation: DataOps introduces automation in repetitive tasks such as data ingestion, cleansing, and transformation. By reducing manual interventions, errors are minimized, and processes are sped up, leading to savings in both time and budget.
Efficient Resource Utilization: With streamlined workflows, resources (both human and computational) are used more effectively. This ensures that expensive data storage and processing infrastructure, as well as data engineers’ and DevOps professionals’ time, are not underutilized or wasted.
Error Reduction: Through continuous monitoring and validation, DataOps reduces errors in data pipelines. This not only prevents costly rework but also avoids decisions made on faulty data, which can have significant financial implications.
Accelerated Time-to-Value: By speeding up data pipelines, businesses can derive insights faster, enabling quicker decision-making and more timely actions in the market, ultimately leading to potential revenue boosts.
ROI from Data: Reduced operational costs directly translate to improved profitability. Moreover, by ensuring high-quality data and faster insights, companies can make better strategic decisions, potentially opening up new revenue streams or identifying cost-saving opportunities.
For example, Alison.ai, an AI-powered video analysis platform, needed an efficient way to manage and consolidate data from various marketing platforms. They were spending hours extracting data for each client. With Rivery’s DataOps engine, Alison.ai established multi-tenant data pipelines, allowing them to customize data retrieval for each client. The platform also automated processes such as client onboarding and data retrieval. As a result, tasks that took hours were reduced to minutes, saving costs and boosting efficiency, eliminating the need for additional data engineers and tools like Airflow.
Scalability and Flexibility
As businesses evolve, so does the volume, velocity, and variety of their data. The ability to scale data operations without skyrocketing costs or compromising on efficiency is essential as businesses grow.
Equally important is the flexibility to adapt to ever-changing data landscapes. DataOps, with its agile and adaptive methodologies, addresses these challenges head-on, providing businesses with the necessary tools to scale and adapt in a dynamic data-driven world.
Scaling Data Operations Efficiently with DataOps
Dynamic Resource Allocation: DataOps emphasizes the use of cloud platforms and containerization technologies like Docker and Kubernetes. These enable dynamic resource allocation, allowing businesses to scale up or down based on their data processing needs.
Parallel Processing: DataOps practices often involve tools and methodologies that support parallel data processing, ensuring large data sets are handled efficiently.
Cost-Effective Scaling: By automating repetitive tasks and optimizing workflows, DataOps ensures that scaling doesn’t necessarily lead to proportionate increases in costs. Businesses can handle more data without linear increases in expenses.
Take, for example, the pharmaceutical giant Bayer. Despite being one of the world’s largest pharmaceutical companies with a revenue of $45bn in 2020, Bayer’s all-in-one marketing tool was limited in data source coverage and lacked transparency and detail. They turned to Rivery, with its 200+ native data connectors, which allowed Bayer to connect instantly to all required sources, including new ones like Twitter and Snapchat. In addition to reformatting existing data for better details, Rivery’s solution integrated seamlessly with Snowflake data warehousing and Tableau dashboards. Within six months of implementation, Bayer’s data usage on the platform grew by 30%.
How Businesses Can Benefit from DataOps: Steps, Strategies, and Best Practices
In the data-driven era, organizations that can harness the power of their data efficiently and effectively are often the ones that stay ahead of the competition. DataOps, with its combination of agile methodologies, technological solutions, and collaborative approaches, can be a game-changer. Here’s how organizations can make the most of it:
Steps and Strategies to Leverage DataOps
Assess Current Data Landscape: Begin by understanding the current data infrastructure, tools, processes, and challenges. A thorough assessment lays the foundation for a tailored DataOps approach.
Define Clear Objectives: Establish clear goals for what you hope to achieve with DataOps – whether it’s faster data processing, improved data quality, better collaboration between teams, or all of the above.
Choose the Right Tools: Invest in tools and platforms that align with your DataOps objectives. This might include data integration platforms, automated testing tools, and monitoring solutions.
Implement Continuous Integration and Continuous Delivery (CI/CD) for Data: Adopt CI/CD principles to ensure your data pipelines are always optimized, adaptable, and up-to-date.
Promote Collaboration: Foster a culture of collaboration between data teams and other departments. Implement regular cross-functional meetings, feedback sessions, and training programs.
Synchronize Goals: Ensure that DataOps initiatives align with broader business goals. For example, if the business objective is to enhance customer experience, DataOps efforts should prioritize data sources and analytics related to customer behavior and feedback.
Establish Feedback Loops: Establish mechanisms to relay insights from data analytics back to decision-makers, ensuring that data-driven insights directly inform business strategies.
Conduct Periodic Review: As business objectives evolve, revisit and recalibrate DataOps strategies to stay aligned.
Best Practices and Actionable Tips
By understanding the potential of DataOps and aligning it with business goals, organizations can unlock unprecedented efficiencies, insights, and advantages in the competitive landscape. The key is a committed approach, continuous learning, and fostering a collaborative, data-centric culture:
Prioritize Data Quality: Always prioritize the quality of data over quantity. Implement data validation, cleaning, and enrichment practices at every step.
Embrace Automation: Automate repetitive tasks, such as data ingestion, transformation, and testing, to improve efficiency and reduce errors.
Stay Updated: The world of DataOps is ever-evolving. Regularly update your skills, tools, and methodologies to stay at the forefront.
Implement Role-based Access Control (RBAC): Ensure that sensitive data is accessed only by authorized personnel, bolstering data security.
Measure and Monitor: Establish key performance indicators (KPIs) related to data operations and monitor them. Regularly assess the ROI of your DataOps initiatives.
Next Steps: Getting Started with DataOps
In an era where data is pivotal to success, can businesses afford to overlook the transformative potential of DataOps? The answer is a resounding NO. For those eager to navigate the data landscape, implementing DataOps isn’t just an option; it’s a strategic imperative.
DataOps starts with data integration. Leverage the Rivery.io data integration platform to seamlessly move your data across platforms and kickstart your DataOps journey. With an intuitive, automated platform that ingests data from diverse sources and delivers it in real-time – to warehouses, dashboards, and more – Rivery takes care of the heavy lifting so you can focus on extracting maximum value from your data.
Minimize the firefighting. Maximize ROI on pipelines.
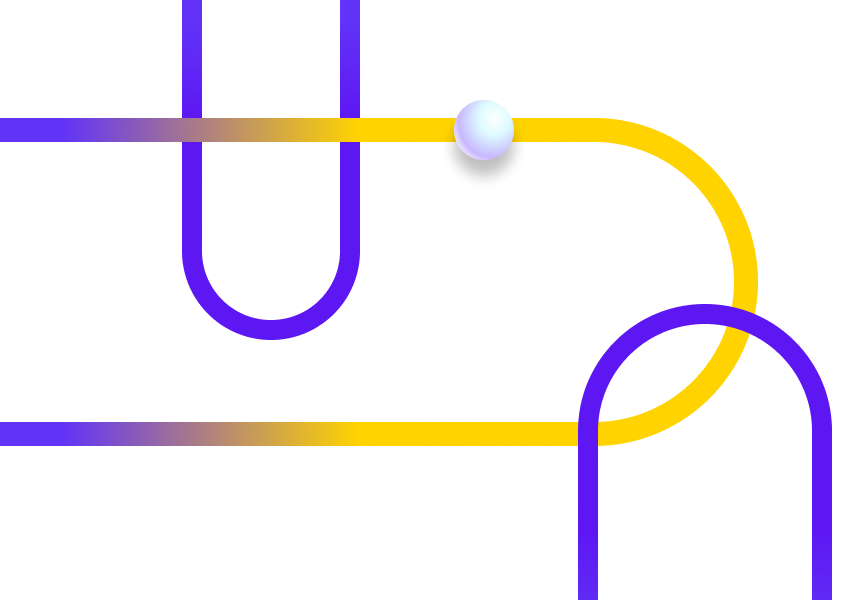
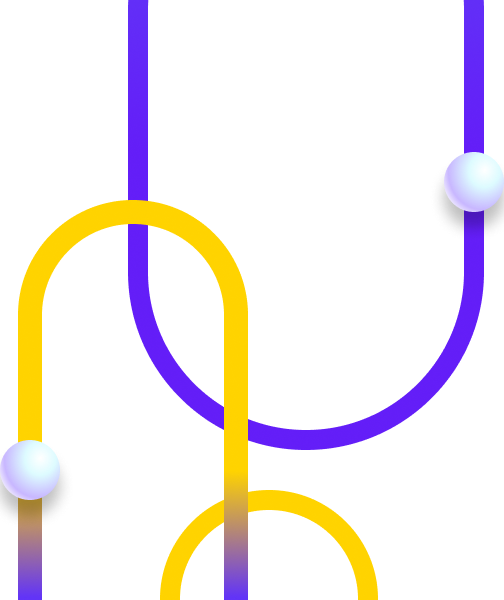